Modeling the marvelous journey from A to B
Assistant Professor Nidhi Seethapathi seeks to understand why we move the way we do.
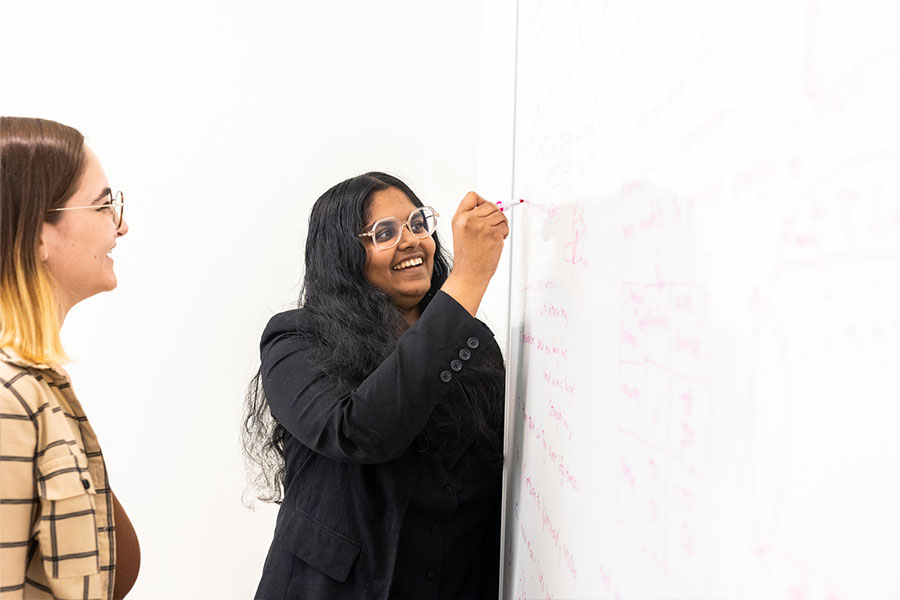
This story originally appeared in the Spring 2023 issue of Spectrum.
___
Nidhi Seethapathi was first drawn to using powerful yet simple models to understand elaborate patterns when she learned about Newton’s laws of motion as a high school student in India. She was fascinated by the idea that wonderfully complex behaviors can arise from a set of objects that follow a few elementary rules.
Now an assistant professor at MIT, Seethapathi seeks to capture the intricacies of movement in the real world, using computational modeling as well as input from theory and experimentation. “[Theoretical physicist and Nobel laureate] Richard Feynman ’39 once said, ‘What I cannot create, I do not understand,’” Seethapathi says. “In that same spirit, the way I try to understand movement is by building models that move the way we do.”
Models of locomotion in the real world
Seethapathi—who holds a shared faculty position between the Department of Brain and Cognitive Sciences and the Department of Electrical Engineering and Computer Science’s Faculty of Artificial Intelligence + Decision- Making, which is housed in the Schwarzman College of Computing and the School of Engineering—recalls a moment during her undergraduate years studying mechanical engineering in Mumbai when a professor asked students to pick an aspect of movement to examine in detail. While most of her peers chose to analyze machines, Seethapathi selected the human hand. She was astounded by its versatility, she says, and by the number of variables, referred to by scientists as “degrees of freedom,” that are needed to characterize routine manual tasks. The assignment made her realize that she wanted to explore the diverse ways in which the entire human body can move.
Also an investigator at the McGovern Institute for Brain Research, Seethapathi pursued graduate research at The Ohio State University Movement Lab, where her goal was to identify the key elements of human locomotion. At that time, most people in the field were analyzing simple movements, she says, “but I was interested in broadening the scope of my models to include real-world behavior. Given that movement is so ubiquitous, I wondered: What can this model say about everyday life?”
After earning her PhD from Ohio State in 2018, Seethapathi continued this line of research as a postdoctoral fellow at the University of Pennsylvania. New computer vision tools to track human movement from video footage had just entered the scene, and during her time at UPenn, Seethapathi sought to expand her skillset to include computer vision and applications to movement rehabilitation.
At MIT, Seethapathi continues to extend the range of her studies of human movement, looking at how locomotion can evolve as people grow and age, and how it can adapt to anatomical changes and even adjust to shifts in weather, which can alter ground conditions. Her investigations now encompass other species as part of an effort to determine how creatures with different morphologies and habitats regulate their movements.
The models Seethapathi and her team create make predictions about human movements that can later be verified or refuted by empirical tests. While relatively simple experiments can be carried out on treadmills, her group is developing measurement systems incorporating wearable sensors and video-based sensing to measure movement data that have traditionally been hard to obtain outside the laboratory.
Although Seethapathi says she is primarily driven to uncover the fundamental principles that govern movement behavior, she believes her work also has practical applications.
“When people are treated for a movement disorder, the goal is to impact their movements in the real world,” she says. “We can use our predictive models to see how a particular intervention will affect a person’s trajectory. The hope is that our models can help put the individual on the right track to recovery as early as possible.”