PhD student Wei-Chen Wang is moved to help people heal
Research draws on simulated robots to illuminate movement—knowledge that might someday help people come back from injury.
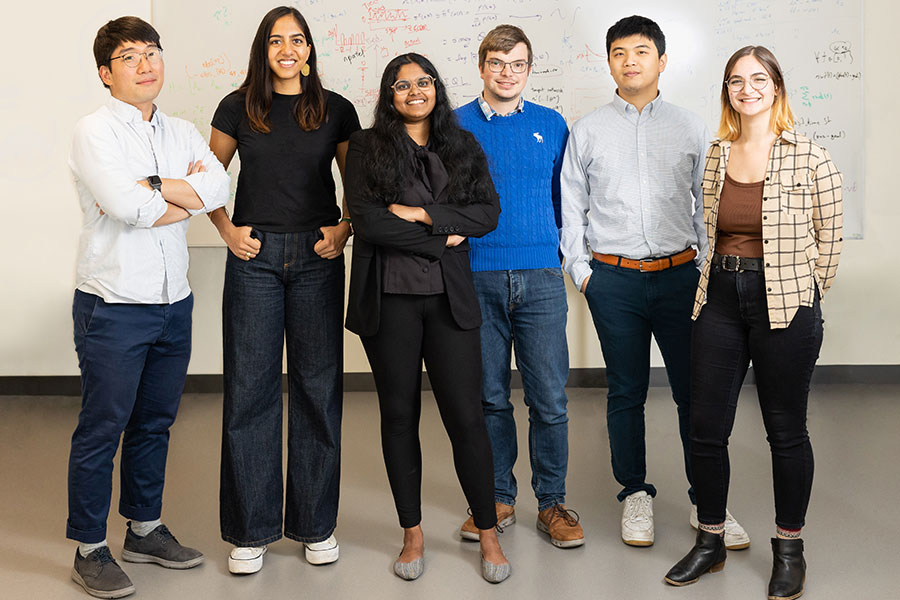
This story originally appeared in the Spring 2023 issue of Spectrum.
___
When he turned his ankle five years ago as an undergraduate playing pickup basketball at the University of Illinois, Wei-Chen (Eric) Wang SM ’22 knew his life would change in certain ways. For one thing, Wang, then a computer science major, wouldn’t be playing basketball anytime soon. He also assumed, correctly, that he might require physical therapy (PT).
What he did not foresee was that this minor injury would influence his career trajectory. While lying on the PT bench, Wang began to wonder: “Can I replicate what the therapist is doing using a robot?” It was an idle thought at the time. Today, however, his research involves robots and movement, closely related to what had seemed a passing fancy.
Wang continued his focus on computer science as an MIT graduate student, receiving his master’s in 2022 before deciding to pursue work of a more applied nature. He met Nidhi Seethapathi, who had joined MIT’s faculty as an assistant professor in electrical engineering and computer science and brain and cognitive science a few months earlier, and was intrigued by the notion of creating robots that could illuminate the key principles of movement—knowledge that might someday help people regain the ability to move comfortably after suffering from injury, stroke, or disease.
As the first PhD student in Seethapathi’s group and a MathWorks Fellow, Wang is charged with building machine learning-based models that can accurately predict and reproduce human movements. He will then use computer-simulated environments to visualize and evaluate the performance of these models.
To begin, he needs to gather data about specific human movements. One potential data collection method involves the placement of sensors or markers on different parts of the body to pinpoint their precise positions at any given moment. He can then try to calculate those positions in the future, as dictated by the equations of motion in physics.
The other method relies on computer vision-powered software that can automatically convert video footage to motion data. Wang prefers the latter approach, which he considers more natural. “We just look at what humans are doing and try to learn from that directly,” he explains. That’s also where machine learning comes in. “We use machine-learning tools to extract data from the video, and those data become the input to our model,” he adds. The model, in this case, is just another term for the robot brain.
The near-term goal is not to make robots more natural, Wang notes. “We’re using [simulated] robots to understand how humans are moving and eventually to explain any kind of movement—or at least that’s the hope. That said, based on the general principles we’re able to abstract, we might someday build robots that can move more naturally.”
Wang is also collaborating on a project headed by postdoctoral fellow Antoine De Comité that focuses on robotic retrieval of objects—the movements required to remove books from a library shelf, for example, or to grab a drink from a refrigerator. While robots routinely excel at tasks such as grasping an object on a tabletop, performing naturalistic movements in three dimensions remains challenging.
Wang describes a video shown by a Stanford University scientist in which a robot destroyed a refrigerator while attempting to extract a beer. He and De Comité hope for better results with robots that have undergone reinforcement learning—an approach using deep learning in which desired motions are rewarded or reinforced whereas unwanted motions are discouraged.
If they succeed in designing a robot that can safely retrieve a beer, Wang says, then more important and delicate tasks could be within reach. Someday, a robot at PT might guide a patient through knee exercises or apply ultrasound to an arthritic elbow.