The McGovern Institute and the Department of Brain and Cognitive Sciences are pleased to announce the appointment of Sven Dorkenwald as an assistant professor starting in January 2026. A trailblazer in the field of computational neuroscience, Dorkenwald is recognized for his leadership in connectomics—an emerging discipline focused on reconstructing and analyzing neural circuitry at unprecedented scale and detail.
“We are thrilled to welcome Sven to MIT” says McGovern Institute Director Robert Desimone. “He brings visionary science and a collaborative spirit to a rapidly advancing area of brain and cognitive sciences and his appointment strengthens MIT’s position at the forefront of brain research.”
Dorkenwald’s research is driven by a bold vision: to develop and apply cutting-edge computational methods that reveal how brain circuits are organized and how they give rise to complex computations. His innovative work has led to transformative advances in the reconstruction of connectomes (detailed neural maps) from nanometer-scale electron microscopy images. He has championed open team science and data sharing and played a central role in producing the first connectome of an entire fruit fly brain—a groundbreaking achievement that is reshaping our understanding of sensory processing and brain circuit function.
“Sven is a rising leader in computational neuroscience who has already made significant contributions toward advancing our understanding of the brain,” says Michale Fee, the Glen V. and Phyllis F. Dorflinger Professor of Neuroscience, and Department Head of Brain and Cognitive Sciences. “He brings a combination of technical expertise, a collaborative mindset, and a strong commitment to open science that will be invaluable to our department. I’m pleased to welcome him to our community and look forward to the impact he will have.”
Dorkenwald earned his BS in physics in 2014 and MS in computer engineering in 2017 from the University of Heidelberg, Germany. He began his research in connectomics as an undergraduate in the group of Winfried Denk at the Max Planck Institute for Medical Research and Max Planck Institute of Neurobiology. Dorkenwald went on to complete his PhD at Princeton University in 2023, where he studied both computer science and neuroscience under the mentorship of Sebastian Seung and Mala Murthy.
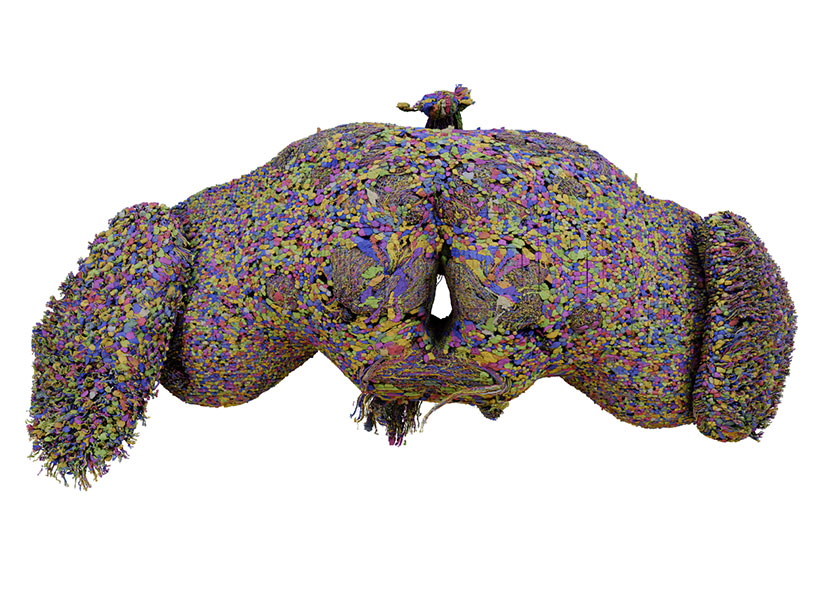
As a PhD student at Princeton, Dorkenwald spearheaded the FlyWire Consortium, a group of more than 200 scientists, gamers, and proofreaders who combined their skills to create the fruit fly connectome. More than 20 million scientific images of the adult fruit fly brain were added to an AI model that traced each neuron and synapse in exquisite detail. Members of the consortium then checked the results produced by the AI model and pieced them together into a complete, three-dimensional map. With over 140,000 neurons, it is the most complex brain completely mapped to date. The findings were published in a special issue of Nature in 2024.
Dorkenwald’s work also played a key role in the MICrONS’ consortium effort to reconstruct a cubic millimeter connectome of the mouse visual cortex. Within the MICrONS effort, he co-lead the development of the software infrastructure, CAVE, that enables scientists to collaboratively edit and analyze large connectomics datasets, including FlyWire’s. The findings of the MICrONS consortium were published in a special issue of Nature in 2025.
Dorkenwald is currently a Shanahan Fellow at the Allen Institute and the University of Washington. He also serves as a visiting faculty researcher at Google Research, where he has been developing machine learning approaches for the annotation of cell reconstructions as part of the Neuromancer team led by Viren Jain.
As an investigator at the McGovern Institute and an assistant professor in the department of brain and cognitive sciences at MIT, Dorkenwald plans to develop computational approaches to overcome challenges in scaling connectomics to whole mammalian brains with the goal of advancing our mechanistic understanding of neuronal circuits and analyzing how they compare across regions and species.