There are many drugs that anesthesiologists can use to induce unconsciousness in patients. Exactly how these drugs cause the brain to lose consciousness has been a longstanding question, but MIT neuroscientists have now answered that question for one commonly used anesthesia drug.
Using a novel technique for analyzing neuron activity, the researchers discovered that the drug propofol induces unconsciousness by disrupting the brain’s normal balance between stability and excitability. The drug causes brain activity to become increasingly unstable, until the brain loses consciousness.
“The brain has to operate on this knife’s edge between excitability and chaos.” – Earl K. Miller
“It’s got to be excitable enough for its neurons to influence one another, but if it gets too excitable, it spins off into chaos. Propofol seems to disrupt the mechanisms that keep the brain in that narrow operating range,” says Earl K. Miller, the Picower Professor of Neuroscience and a member of MIT’s Picower Institute for Learning and Memory.
The new findings, reported today in Neuron, could help researchers develop better tools for monitoring patients as they undergo general anesthesia.
Miller and Ila Fiete, a professor of brain and cognitive sciences, the director of the K. Lisa Yang Integrative Computational Neuroscience Center (ICoN), and a member of MIT’s McGovern Institute for Brain Research, are the senior authors of the new study. MIT graduate student Adam Eisen and MIT postdoc Leo Kozachkov are the lead authors of the paper.
Losing consciousness
Propofol is a drug that binds to GABA receptors in the brain, inhibiting neurons that have those receptors. Other anesthesia drugs act on different types of receptors, and the mechanism for how all of these drugs produce unconsciousness is not fully understood.
Miller, Fiete, and their students hypothesized that propofol, and possibly other anesthesia drugs, interfere with a brain state known as “dynamic stability.” In this state, neurons have enough excitability to respond to new input, but the brain is able to quickly regain control and prevent them from becoming overly excited.
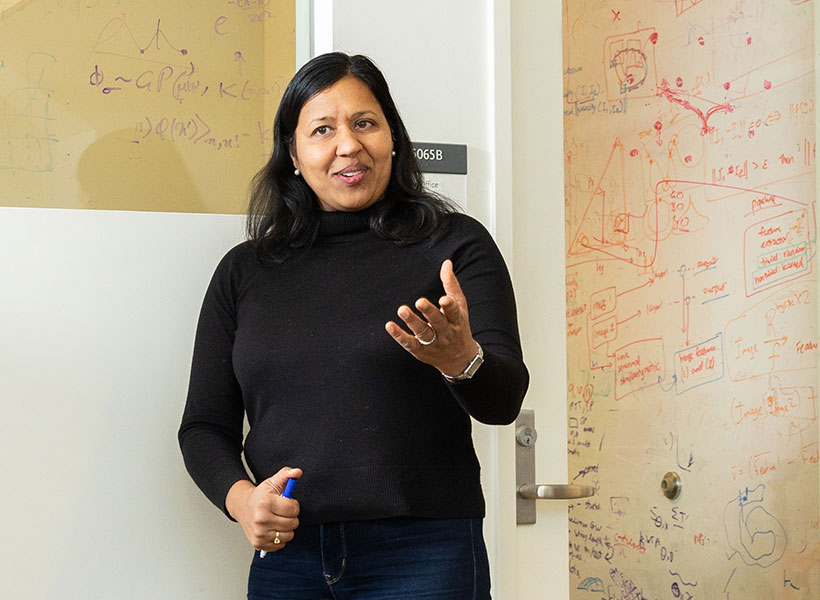
Previous studies of how anesthesia drugs affect this balance have found conflicting results: Some suggested that during anesthesia, the brain shifts toward becoming too stable and unresponsive, which leads to loss of consciousness. Others found that the brain becomes too excitable, leading to a chaotic state that results in unconsciousness.
Part of the reason for these conflicting results is that it has been difficult to accurately measure dynamic stability in the brain. Measuring dynamic stability as consciousness is lost would help researchers determine if unconsciousness results from too much stability or too little stability.
In this study, the researchers analyzed electrical recordings made in the brains of animals that received propofol over an hour-long period, during which they gradually lost consciousness. The recordings were made in four areas of the brain that are involved in vision, sound processing, spatial awareness, and executive function.
These recordings covered only a tiny fraction of the brain’s overall activity, so to overcome that, the researchers used a technique called delay embedding. This technique allows researchers to characterize dynamical systems from limited measurements by augmenting each measurement with measurements that were recorded previously.
Using this method, the researchers were able to quantify how the brain responds to sensory inputs, such as sounds, or to spontaneous perturbations of neural activity.
In the normal, awake state, neural activity spikes after any input, then returns to its baseline activity level. However, once propofol dosing began, the brain started taking longer to return to its baseline after these inputs, remaining in an overly excited state. This effect became more and more pronounced until the animals lost consciousness.
This suggests that propofol’s inhibition of neuron activity leads to escalating instability, which causes the brain to lose consciousness, the researchers say.
Better anesthesia control
To see if they could replicate this effect in a computational model, the researchers created a simple neural network. When they increased the inhibition of certain nodes in the network, as propofol does in the brain, network activity became destabilized, similar to the unstable activity the researchers saw in the brains of animals that received propofol.
“We looked at a simple circuit model of interconnected neurons, and when we turned up inhibition in that, we saw a destabilization. So, one of the things we’re suggesting is that an increase in inhibition can generate instability, and that is subsequently tied to loss of consciousness,” Eisen says.
As Fiete explains, “This paradoxical effect, in which boosting inhibition destabilizes the network rather than silencing or stabilizing it, occurs because of disinhibition. When propofol boosts the inhibitory drive, this drive inhibits other inhibitory neurons, and the result is an overall increase in brain activity.”
The researchers suspect that other anesthetic drugs, which act on different types of neurons and receptors, may converge on the same effect through different mechanisms — a possibility that they are now exploring.
If this turns out to be true, it could be helpful to the researchers’ ongoing efforts to develop ways to more precisely control the level of anesthesia that a patient is experiencing. These systems, which Miller is working on with Emery Brown, the Edward Hood Taplin Professor of Medical Engineering at MIT, work by measuring the brain’s dynamics and then adjusting drug dosages accordingly, in real-time.
“If you find common mechanisms at work across different anesthetics, you can make them all safer by tweaking a few knobs, instead of having to develop safety protocols for all the different anesthetics one at a time,” Miller says. “You don’t want a different system for every anesthetic they’re going to use in the operating room. You want one that’ll do it all.”
The researchers also plan to apply their technique for measuring dynamic stability to other brain states, including neuropsychiatric disorders.
“This method is pretty powerful, and I think it’s going to be very exciting to apply it to different brain states, different types of anesthetics, and also other neuropsychiatric conditions like depression and schizophrenia,” Fiete says.
The research was funded by the Office of Naval Research, the National Institute of Mental Health, the National Institute of Neurological Disorders and Stroke, the National Science Foundation Directorate for Computer and Information Science and Engineering, the Simons Center for the Social Brain, the Simons Collaboration on the Global Brain, the JPB Foundation, the McGovern Institute, and the Picower Institute.