Machine learning can predict bipolar disorder in children and teens
A machine-learning-driven risk calculator could alert health care providers when young people are at risk of developing bipolar disorder.
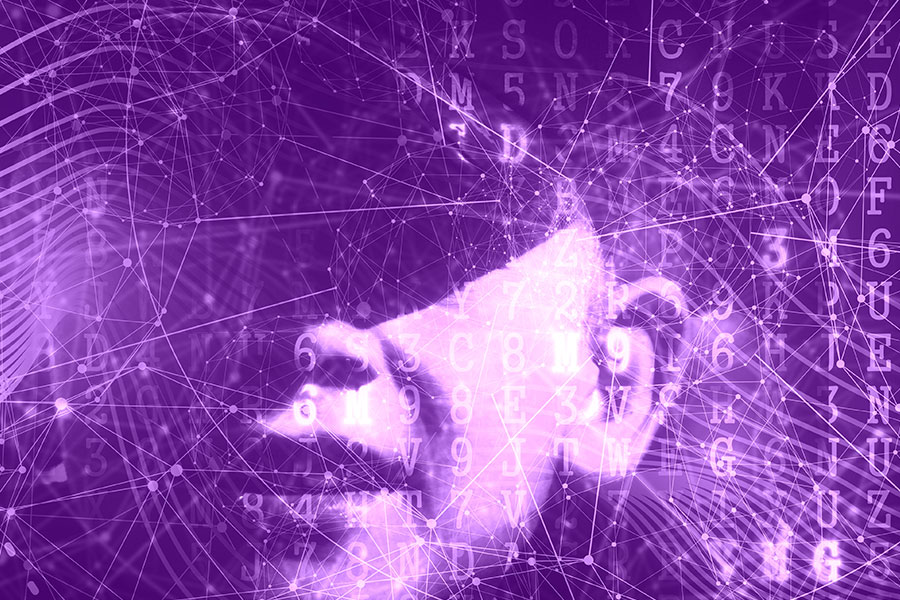
Bipolar disorder often begins in childhood or adolescence, triggering dramatic mood shifts and intense emotions that cause problems at home and school. But the condition is often overlooked or misdiagnosed until patients are older. New research suggests that machine learning, a type of artificial intelligence, could help by identifying children who are at risk of bipolar disorder so doctors are better prepared to recognize the condition if it develops.
On October 13, 2022, researchers led by McGovern Institute investigator John Gabrieli and collaborators at Massachusetts General Hospital reported in the Journal of Psychiatric Research that when presented with clinical data on nearly 500 children and teenagers, a machine learning model was able to identify about 75 percent of those who were later diagnosed with bipolar disorder. The approach performs better than any other method of predicting bipolar disorder, and could be used to develop a simple risk calculator for health care providers.
Gabrieli says such a tool would be particularly valuable because bipolar disorder is less common in children than conditions like major depression, with which it shares symptoms, and attention-deficit/ hyperactivity disorder (ADHD), with which it often co-occurs. “Humans are not well tuned to watch out for rare events,” he says. “If you have a decent measure, it’s so much easier for a machine to identify than humans. And in this particular case, [the machine learning prediction] was surprisingly robust.”
Detecting bipolar disorder
Mai Uchida, Director of Massachusetts General Hospital’s Child Depression Program, says that nearly two percent of youth worldwide are estimated to have bipolar disorder, but diagnosing pediatric bipolar disorder can be challenging. A certain amount of emotional turmoil is to be expected in children and teenagers, and even when moods become seriously disruptive, children with bipolar disorder are often initially diagnosed with major depression or ADHD. That’s a problem, because the medications used to treat those conditions often worsen the symptoms of bipolar disorder. Tailoring treatment to a diagnosis of bipolar disorder, in contrast, can lead to significant improvements for patients and their families. “When we can give them a little bit of ease and give them a little bit of control over themselves, it really goes a long way,” Uchida says.
In fact, a poor response to antidepressants or ADHD medications can help point a psychiatrist toward a diagnosis of bipolar disorder. So too can a child’s family history, in addition to their own behavior and psychiatric history. But, Uchida says, “it’s kind of up to the individual clinician to pick up on these things.”
Uchida and Gabrieli wondered whether machine learning, which can find patterns in large, complex datasets, could focus in on the most relevant features to identify individuals with bipolar disorder. To find out, they turned to data from a study that began in the 1990s. The study, headed by Joseph Biederman, Chief of the Clinical and Research Programs in Pediatric Psychopharmacology and Adult ADHD at Massachusetts General Hospital, had collected extensive psychiatric assessments of hundreds of children with and without ADHD, then followed those individuals for ten years.
To explore whether machine learning could find predictors of bipolar disorder within that data, Gabrieli, Uchida, and colleagues focused on 492 children and teenagers without ADHD, who were recruited to the study as controls. Over the ten years of the study, 45 of those individuals developed bipolar disorder.
Within the data collected at the study’s outset, the machine learning model was able to find patterns that associated with a later diagnosis of bipolar disorder. A few behavioral measures turned out to be particularly relevant to the model’s predictions: children and teens with combined problems with attention, aggression, and anxiety were most likely to later be diagnosed with bipolar disorder. These indicators were all picked up by a standard assessment tool called the Child Behavior Checklist.
Uchida and Gabrieli say the machine learning model could be integrated into the medical record system to help pediatricians and child psychiatrists catch early warning signs of bipolar disorder. “The information that’s collected could alert a clinician to the possibility of a bipolar disorder developing,” Uchida says. “Then at least they’re aware of the risk, and they may be able to maybe pick up on some of the deterioration when it’s happening and think about either referring them or treating it themselves.”