“It’s amazing to me that a tiny worm — with neither eyes nor the molecular machinery used by eyes to detect colors — can identify and avoid a toxic bacterium based, in part, on its blue color,” says H. Robert Horvitz, the David H. Koch Professor of Biology at MIT, a member of the McGovern Institute for Brain Research and the Koch Institute for Integrative Cancer Research, Howard Hughes Medical Institute Investigator, and the co-senior author of the study.
“One of the joys of being a biologist is the opportunity to discover things about nature that no one has ever imagined before,” says Horvitz.
A model organism
The roundworm in question, Caenorhabditis elegans, is only about a millimeter long. Despite their minute stature and simple nervous system, these nematodes display a complex repertoire of behaviors. They can smell, taste, sense touch, react to temperature, and even escape or change their feeding patterns in response to bright, blue light. Although researchers once thought that these worms bury themselves deep in soil, it’s becoming increasingly clear that C. elegans prefers compost heaps above ground that offer some sun exposure. As a result, roundworms may have a need for light- and color-sensing capabilities after all.
The decomposing organic matter where C. elegans resides offers an array of scrumptious microbes, including bacteria like Pseudomonas aeruginosa, which secretes a distinctive blue toxin. Previous studies showed that worms in the lab feed on a lawn of P. aeruginosa for a few hours and then begin avoiding their food — perhaps because the bacteria continue to divide and excrete more of the colorful poison. Dipon Ghosh, Horvitz lab postdoc and the study’s first author, wondered whether the worms were using the distinctive color to determine if their meal was too toxic to consume.
A spectrum of behavior
Over the course of his experiments, Ghosh noticed that his worms were more likely to flee the colorful bacterial lawn if it was bathed in white light from a nearby LED bulb. This finding was curious on its own, but Ghosh wanted know if the blue toxin played a role as well.
To test this theory, he first exchanged the blue toxin for a harmless dye of the same color, and then for a clear, colorless toxin. On its own, neither substitute was sufficient to spur avoidance. Only together did they prompt a response — suggesting the worms were assessing both the toxic nature and the color of the P. aeruginosa secretions simultaneously. Once again, this behavioral pattern only emerged in the presence of the LED’s white light.
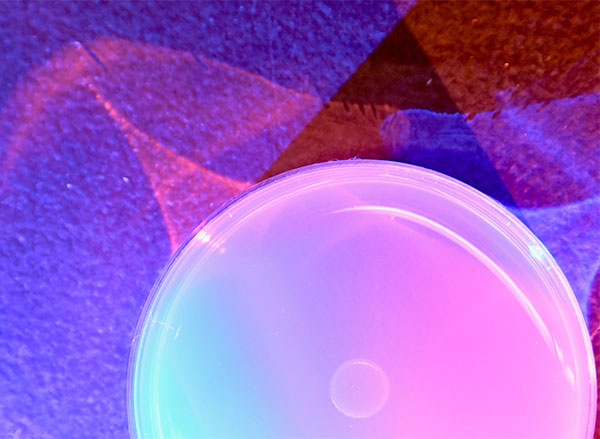
Intrigued, Ghosh wanted to examine what it was about the blue color that triggered avoidance. This time, he used two colored LED lights, one blue and one amber, to tint the ambient light. In doing so, he could control the ratio of wavelengths without changing the total energy delivered to the worms. The beam had previously contained the entire visible spectrum, but mixing the amber and blue bulbs allowed Ghosh to tweak the relative amounts of short-wavelength blue light and long-wavelength amber light. Surprisingly, the worms only fled the bacterial lawn when their environment was bathed in light with specific blue:amber ratios.
“We were able to definitively show that worms aren’t sensing the world in grayscale and simply evaluating the levels of brightness and darkness,” Ghosh says. “They’re actually comparing ratios of wavelengths and using that information to make decisions — which was thoroughly unexpected.”
It wasn’t until Ghosh ran his experiments again, this time using various types of wild C. elegans, that he realized the popular laboratory strain he’d been using was actually less color-sensitive compared to its close relatives. After analyzing the genomes of these worms, he was able to identify two genes in particular (called jkk-1 and lec-3) that contributed to these variations in color-dependent foraging.
Although the two genes play many important functions in a variety of organisms, including humans, they are both involved in molecular pathways that help cells respond to stress caused by damaging ultraviolet light.
“We’ve discovered that the color of light in the worm’s environment can influence how the worm navigates the world,” Ghosh says. “But our work suggests that many genes, in addition to the two we’ve already identified, can affect color sensitivity, and we’re now exploring how.”
Nature’s innovation
The notion that worms can sense color is “astounding” and showcases nature’s innovation, according to Leslie Vosshall, Robin Chemers Neustein Professor and Howard Hughes Medical Institute Investigator at The Rockefeller University, who was not involved in the study. “These worms are sliding around in a dim muck with colorful, toxic bacteria. It would be helpful to see and avoid them, so the worms somehow evolved a completely new way to see.”
Vosshall is curious about which cells in C. elegans help discriminate light, as well as the specific roles that the jkk-1 and lec-3 genes play in mediating light perception. “This paper, like all important papers, raises many additional questions,” she says.
Ghosh suspects the lab’s findings could generalize to other critters besides roundworms. If nothing else, it’s clear that light-sensitivity does not always require vision — or eyes. C. elegans are seeing the light, and now so are the biologists.
This research was funded by the Howard Hughes Medical Institute and National Institute of General Medical Sciences.