Having trouble paying attention? MIT neuroscientists may have a solution for you: Turn down your alpha brain waves. In a new study, the researchers found that people can enhance their attention by controlling their own alpha brain waves based on neurofeedback they receive as they perform a particular task.
The study found that when subjects learned to suppress alpha waves in one hemisphere of their parietal cortex, they were able to pay better attention to objects that appeared on the opposite side of their visual field. This is the first time that this cause-and-effect relationship has been seen, and it suggests that it may be possible for people to learn to improve their attention through neurofeedback.
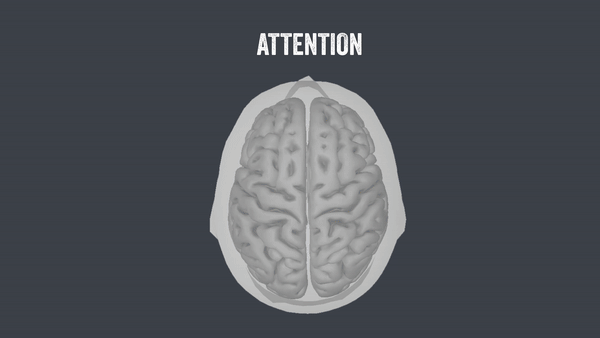
“There’s a lot of interest in using neurofeedback to try to help people with various brain disorders and behavioral problems,” says Robert Desimone, director of MIT’s McGovern Institute for Brain Research. “It’s a completely noninvasive way of controlling and testing the role of different types of brain activity.”
It’s unknown how long these effects might last and whether this kind of control could be achieved with other types of brain waves, such as beta waves, which are linked to Parkinson’s disease. The researchers are now planning additional studies of whether this type of neurofeedback training might help people suffering from attentional or other neurological disorders.
Desimone is the senior author of the paper, which appears in Neuron on Dec. 4. McGovern Institute postdoc Yasaman Bagherzadeh is the lead author of the study. Daniel Baldauf, a former McGovern Institute research scientist, and Dimitrios Pantazis, a McGovern Institute principal research scientist, are also authors of the paper.
Alpha and attention
There are billions of neurons in the brain, and their combined electrical signals generate oscillations known as brain waves. Alpha waves, which oscillate in the frequency of 8 to 12 hertz, are believed to play a role in filtering out distracting sensory information.
Previous studies have shown a strong correlation between attention and alpha brain waves, particularly in the parietal cortex. In humans and in animal studies, a decrease in alpha waves has been linked to enhanced attention. However, it was unclear if alpha waves control attention or are just a byproduct of some other process that governs attention, Desimone says.
To test whether alpha waves actually regulate attention, the researchers designed an experiment in which people were given real-time feedback on their alpha waves as they performed a task. Subjects were asked to look at a grating pattern in the center of a screen, and told to use mental effort to increase the contrast of the pattern as they looked at it, making it more visible.
During the task, subjects were scanned using magnetoencephalography (MEG), which reveals brain activity with millisecond precision. The researchers measured alpha levels in both the left and right hemispheres of the parietal cortex and calculated the degree of asymmetry between the two levels. As the asymmetry between the two hemispheres grew, the grating pattern became more visible, offering the participants real-time feedback.
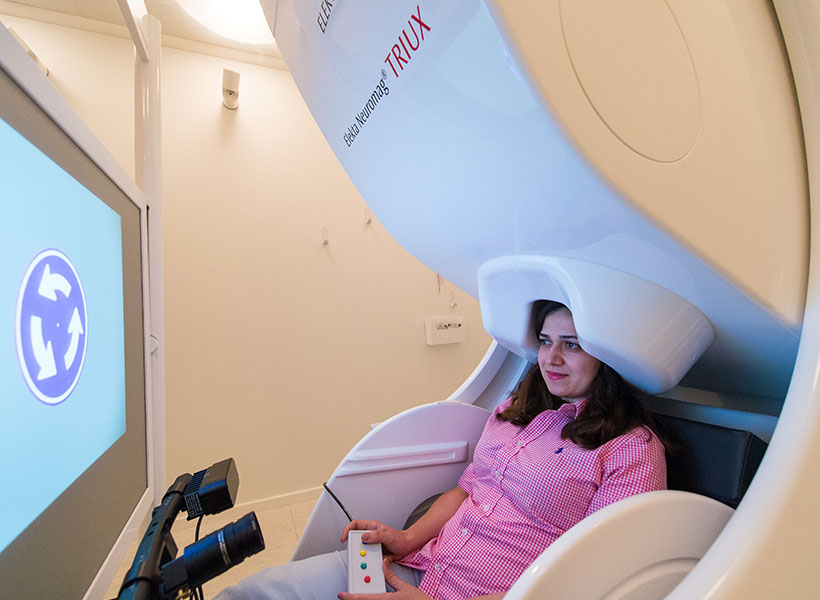
Although subjects were not told anything about what was happening, after about 20 trials (which took about 10 minutes), they were able to increase the contrast of the pattern. The MEG results indicated they had done so by controlling the asymmetry of their alpha waves.
“After the experiment, the subjects said they knew that they were controlling the contrast, but they didn’t know how they did it,” Bagherzadeh says. “We think the basis is conditional learning — whenever you do a behavior and you receive a reward, you’re reinforcing that behavior. People usually don’t have any feedback on their brain activity, but when we provide it to them and reward them, they learn by practicing.”
Although the subjects were not consciously aware of how they were manipulating their brain waves, they were able to do it, and this success translated into enhanced attention on the opposite side of the visual field. As the subjects looked at the pattern in the center of the screen, the researchers flashed dots of light on either side of the screen. The participants had been told to ignore these flashes, but the researchers measured how their visual cortex responded to them.
One group of participants was trained to suppress alpha waves in the left side of the brain, while the other was trained to suppress the right side. In those who had reduced alpha on the left side, their visual cortex showed a larger response to flashes of light on the right side of the screen, while those with reduced alpha on the right side responded more to flashes seen on the left side.
“Alpha manipulation really was controlling people’s attention, even though they didn’t have any clear understanding of how they were doing it,” Desimone says.
Persistent effect
After the neurofeedback training session ended, the researchers asked subjects to perform two additional tasks that involve attention, and found that the enhanced attention persisted. In one experiment, subjects were asked to watch for a grating pattern, similar to what they had seen during the neurofeedback task, to appear. In some of the trials, they were told in advance to pay attention to one side of the visual field, but in others, they were not given any direction.
When the subjects were told to pay attention to one side, that instruction was the dominant factor in where they looked. But if they were not given any cue in advance, they tended to pay more attention to the side that had been favored during their neurofeedback training.
In another task, participants were asked to look at an image such as a natural outdoor scene, urban scene, or computer-generated fractal shape. By tracking subjects’ eye movements, the researchers found that people spent more time looking at the side that their alpha waves had trained them to pay attention to.
“It is promising that the effects did seem to persist afterwards,” says Desimone, though more study is needed to determine how long these effects might last.
The research was funded by the McGovern Institute.