When listening to music, the human brain appears to be biased toward hearing and producing rhythms composed of simple integer ratios — for example, a series of four beats separated by equal time intervals (forming a 1:1:1 ratio).
However, the favored ratios can vary greatly between different societies, according to a large-scale study led by researchers at MIT and the Max Planck Institute for Empirical Aesthetics and carried out in 15 countries. The study included 39 groups of participants, many of whom came from societies whose traditional music contains distinctive patterns of rhythm not found in Western music.
“Our study provides the clearest evidence yet for some degree of universality in music perception and cognition, in the sense that every single group of participants that was tested exhibits biases for integer ratios. It also provides a glimpse of the variation that can occur across cultures, which can be quite substantial,” says Nori Jacoby, the study’s lead author and a former MIT postdoc, who is now a research group leader at the Max Planck Institute for Empirical Aesthetics in Frankfurt, Germany.
The brain’s bias toward simple integer ratios may have evolved as a natural error-correction system that makes it easier to maintain a consistent body of music, which human societies often use to transmit information.
“When people produce music, they often make small mistakes. Our results are consistent with the idea that our mental representation is somewhat robust to those mistakes, but it is robust in a way that pushes us toward our preexisting ideas of the structures that should be found in music,” says Josh McDermott, an associate professor of brain and cognitive sciences at MIT and a member of MIT’s McGovern Institute for Brain Research and Center for Brains, Minds, and Machines.
McDermott is the senior author of the study, which appears today in Nature Human Behaviour. The research team also included scientists from more than two dozen institutions around the world.
A global approach
The new study grew out of a smaller analysis that Jacoby and McDermott published in 2017. In that paper, the researchers compared rhythm perception in groups of listeners from the United States and the Tsimane’, an Indigenous society located in the Bolivian Amazon rainforest.
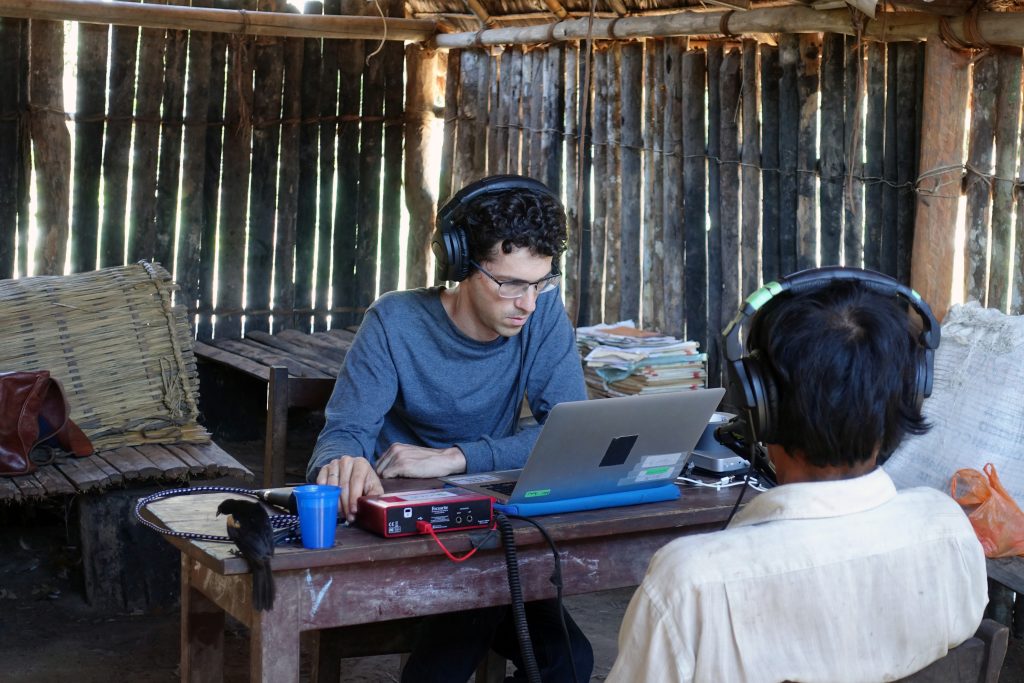
To measure how people perceive rhythm, the researchers devised a task in which they play a randomly generated series of four beats and then ask the listener to tap back what they heard. The rhythm produced by the listener is then played back to the listener, and they tap it back again. Over several iterations, the tapped sequences became dominated by the listener’s internal biases, also known as priors.
“The initial stimulus pattern is random, but at each iteration the pattern is pushed by the listener’s biases, such that it tends to converge to a particular point in the space of possible rhythms,” McDermott says. “That can give you a picture of what we call the prior, which is the set of internal implicit expectations for rhythms that people have in their heads.”
When the researchers first did this experiment, with American college students as the test subjects, they found that people tended to produce time intervals that are related by simple integer ratios. Furthermore, most of the rhythms they produced, such as those with ratios of 1:1:2 and 2:3:3, are commonly found in Western music.
The researchers then went to Bolivia and asked members of the Tsimane’ society to perform the same task. They found that Tsimane’ also produced rhythms with simple integer ratios, but their preferred ratios were different and appeared to be consistent with those that have been documented in the few existing records of Tsimane’ music.
“At that point, it provided some evidence that there might be very widespread tendencies to favor these small integer ratios, and that there might be some degree of cross-cultural variation. But because we had just looked at this one other culture, it really wasn’t clear how this was going to look at a broader scale,” Jacoby says.
To try to get that broader picture, the MIT team began seeking collaborators around the world who could help them gather data on a more diverse set of populations. They ended up studying listeners from 39 groups, representing 15 countries on five continents — North America, South America, Europe, Africa, and Asia.
“This is really the first study of its kind in the sense that we did the same experiment in all these different places, with people who are on the ground in those locations,” McDermott says. “That hasn’t really been done before at anything close to this scale, and it gave us an opportunity to see the degree of variation that might exist around the world.”
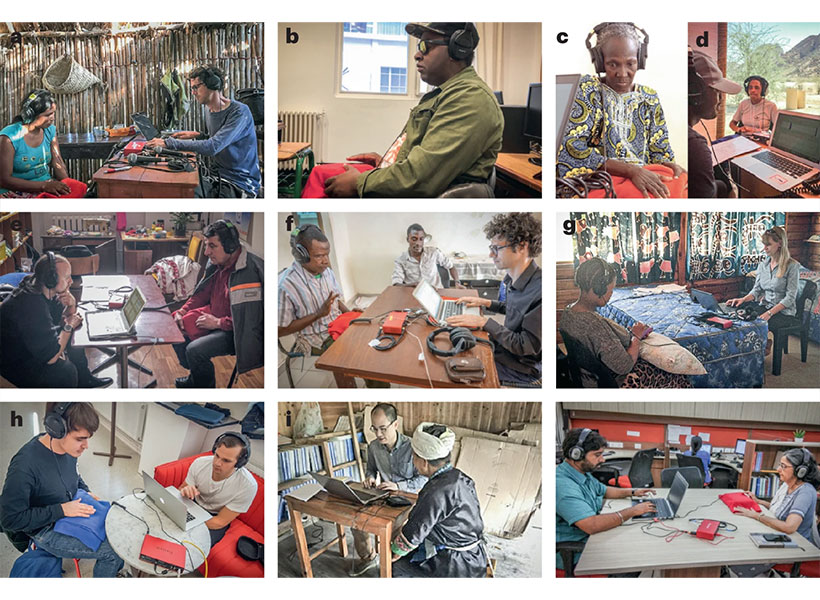
Cultural comparisons
Just as they had in their original 2017 study, the researchers found that in every group they tested, people tended to be biased toward simple integer ratios of rhythm. However, not every group showed the same biases. People from North America and Western Europe, who have likely been exposed to the same kinds of music, were more likely to generate rhythms with the same ratios. However, many groups, for example those in Turkey, Mali, Bulgaria, and Botswana showed a bias for other rhythms.
“There are certain cultures where there are particular rhythms that are prominent in their music, and those end up showing up in the mental representation of rhythm,” Jacoby says.
The researchers believe their findings reveal a mechanism that the brain uses to aid in the perception and production of music.
“When you hear somebody playing something and they have errors in their performance, you’re going to mentally correct for those by mapping them onto where you implicitly think they ought to be,” McDermott says. “If you didn’t have something like this, and you just faithfully represented what you heard, these errors might propagate and make it much harder to maintain a musical system.”
Among the groups that they studied, the researchers took care to include not only college students, who are easy to study in large numbers, but also people living in traditional societies, who are more difficult to reach. Participants from those more traditional groups showed significant differences from college students living in the same countries, and from people who live in those countries but performed the test online.
“What’s very clear from the paper is that if you just look at the results from undergraduate students around the world, you vastly underestimate the diversity that you see otherwise,” Jacoby says. “And the same was true of experiments where we tested groups of people online in Brazil and India, because you’re dealing with people who have internet access and presumably have more exposure to Western music.”
The researchers now hope to run additional studies of different aspects of music perception, taking this global approach.
“If you’re just testing college students around the world or people online, things look a lot more homogenous. I think it’s very important for the field to realize that you actually need to go out into communities and run experiments there, as opposed to taking the low-hanging fruit of running studies with people in a university or on the internet,” McDermott says.
The research was funded by the James S. McDonnell Foundation, the Canadian National Science and Engineering Research Council, the South African National Research Foundation, the United States National Science Foundation, the Chilean National Research and Development Agency, the Austrian Academy of Sciences, the Japan Society for the Promotion of Science, the Keio Global Research Institute, the United Kingdom Arts and Humanities Research Council, the Swedish Research Council, and the John Fell Fund.