This story originally appeared in the Spring 2023 issue of BrainScan.
___
Artificial intelligence seems to have gotten a lot smarter recently. AI technologies are increasingly integrated into our lives — improving our weather forecasts, finding efficient routes through traffic, personalizing the ads we see and our experiences with social media.
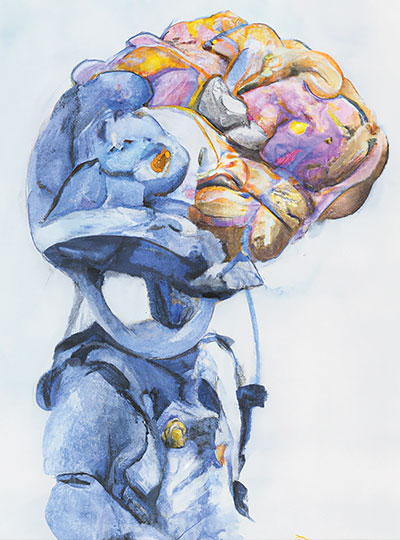
But with the debut of powerful new chatbots like ChatGPT, millions of people have begun interacting with AI tools that seem convincingly human-like. Neuroscientists are taking note — and beginning to dig into what these tools tell us about intelligence and the human brain.
The essence of human intelligence is hard to pin down, let alone engineer. McGovern scientists say there are many kinds of intelligence, and as humans, we call on many different kinds of knowledge and ways of thinking. ChatGPT’s ability to carry on natural conversations with its users has led some to speculate the computer model is sentient, but McGovern neuroscientists insist that the AI technology cannot think for itself.
Still, they say, the field may have reached a turning point.
“I still don’t believe that we can make something that is indistinguishable from a human. I think we’re a long way from that. But for the first time in my life I think there is a small, nonzero chance that it may happen in the next year,” says McGovern founding member Tomaso Poggio, who has studied both human intelligence and machine learning for more than 40 years.
Different sort of intelligence
Developed by the company OpenAI, ChatGPT is an example of a deep neural network, a type of machine learning system that has made its way into virtually every aspect of science and technology. These models learn to perform various tasks by identifying patterns in large datasets. ChatGPT works by scouring texts and detecting and replicating the ways language is used. Drawing on language patterns it finds across the internet, ChatGPT can design you a meal plan, teach you about rocket science, or write a high school-level essay about Mark Twain. With all of the internet as a training tool, models like this have gotten so good at what they do, they can seem all-knowing.
“Engineers have been inventing some of these forms of intelligence since the beginning of the computers. ChatGPT is one. But it is very far from human intelligence.” – Tomaso Poggio
Nonetheless, language models have a restricted skill set. Play with ChatGPT long enough and it will surely give you some wrong information, even if its fluency makes its words deceptively convincing. “These models don’t know about the world, they don’t know about other people’s mental states, they don’t know how things are beyond whatever they can gather from how words go together,” says Postdoctoral Associate Anna Ivanova, who works with McGovern Investigators Evelina Fedorenko and Nancy Kanwisher as well as Jacob Andreas in MIT’s Computer Science and Artificial Intelligence Laboratory.
Such a model, the researchers say, cannot replicate the complex information processing that happens in the human brain. That doesn’t mean language models can’t be intelligent — but theirs is a different sort of intelligence than our own. “I think that there is an infinite number of different forms of intelligence,” says Poggio. “Engineers have been inventing some of these forms of intelligence since the beginning of the computers. ChatGPT is one. But it is very far from human intelligence.”
Under the hood
Just as there are many forms of intelligence, there are also many types of deep learning models — and McGovern researchers are studying the internals of these models to better understand the human brain.
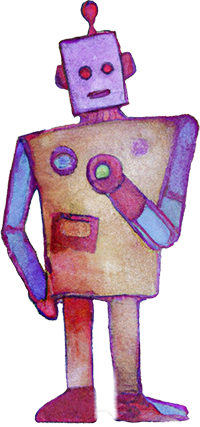
“These AI models are, in a way, computational hypotheses for what the brain is doing,” Kanwisher says. “Up until a few years ago, we didn’t really have complete computational models of what might be going on in language processing or vision. Once you have a way of generating actual precise models and testing them against real data, you’re kind of off and running in a way that we weren’t ten years ago.”
Artificial neural networks echo the design of the brain in that they are made of densely interconnected networks of simple units that organize themselves — but Poggio says it’s not yet entirely clear how they work.
No one expects that brains and machines will work in exactly the same ways, though some types of deep learning models are more humanlike in their internals than others. For example, a computer vision model developed by McGovern Investigator James DiCarlo responds to images in ways that closely parallel the activity in the visual cortex of animals who are seeing the same thing. DiCarlo’s team can even use their model’s predictions to create an image that will activate specific neurons in an animal’s brain.
“We shouldn’t just automatically assume that if we trained a deep network on a task, that it’s going to look like the brain.” – Ila Fiete
Still, there is reason to be cautious in interpreting what artificial neural networks tell us about biology. “We shouldn’t just automatically assume that if we trained a deep network on a task, that it’s going to look like the brain,” says McGovern Associate Investigator Ila Fiete. Fiete acknowledges that it’s tempting to think of neural networks as models of the brain itself due to their architectural similarities — but she says so far, that idea remains largely untested.
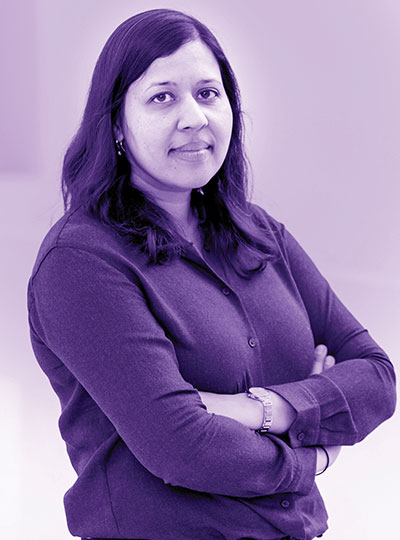
She and her colleagues recently experimented with neural networks that estimate an object’s position in space by integrating information about its changing velocity.
In the brain, specialized neurons known as grid cells carry out this calculation, keeping us aware of where we are as we move through the world. Other researchers had reported that not only can neural networks do this successfully, those that do include components that behave remarkably like grid cells. They had argued that the need to do this kind of path integration must be the reason our brains have grid cells — but Fiete’s team found that artificial networks don’t need to mimic the brain to accomplish this brain-like task. They found that many neural networks can solve the same problem without grid cell-like elements.
One way investigators might generate deep learning models that do work like the brain is to give them a problem that is so complex that there is only one way of solving it, Fiete says.
Language, she acknowledges, might be that complex.
“This is clearly an example of a super-rich task,” she says. “I think on that front, there is a hope that they’re solving such an incredibly difficult task that maybe there is a sense in which they mirror the brain.”
Language parallels
In Fedorenko’s lab, where researchers are focused on identifying and understanding the brain’s language processing circuitry, they have found that some language models do, in fact, mimic certain aspects of human language processing. Many of the most effective models are trained to do a single task: make predictions about word use. That’s what your phone is doing when it suggests words for your text message as you type. Models that are good at this, it turns out, can apply this skill to carrying on conversations, composing essays, and using language in other useful ways. Neuroscientists have found evidence that humans, too, rely on word prediction as a part of language processing.
Fedorenko and her team compared the activity of language models to the brain activity of people as they read or listened to words, sentences, and stories, and found that some models were a better match to human neural responses than others. “The models that do better on this relatively unsophisticated task — just guess what comes next — also do better at capturing human neural responses,” Fedorenko says.
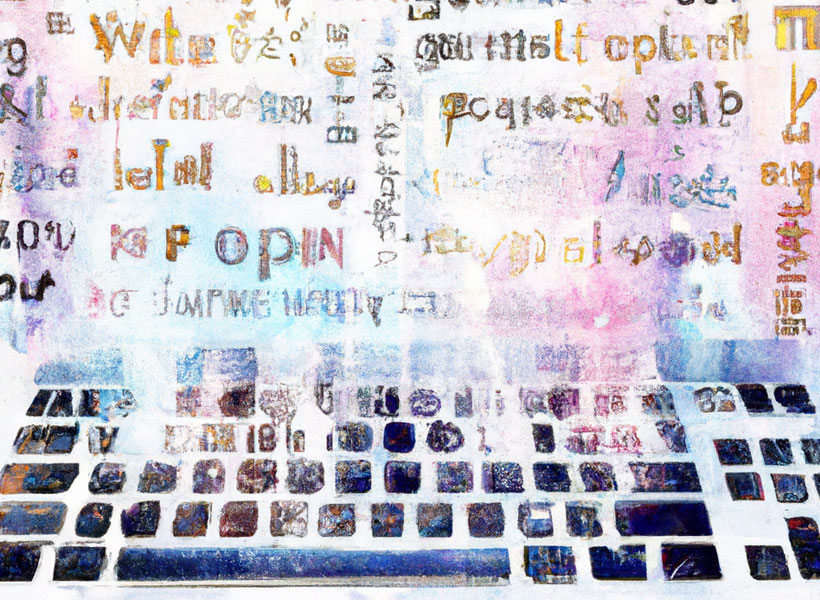
It’s a compelling parallel, suggesting computational models and the human brain may have arrived at a similar solution to a problem, even in the face of the biological constraints that have shaped the latter. For Fedorenko and her team, it’s sparked new ideas that they will explore, in part, by modifying existing language models — possibly to more closely mimic the brain.
With so much still unknown about how both human and artificial neural networks learn, Fedorenko says it’s hard to predict what it will take to make language models work and behave more like the human brain. One possibility they are exploring is training a model in a way that more closely mirrors the way children learn language early in life.
Another question, she says, is whether language models might behave more like humans if they had a more limited recall of their own conversations. “All of the state-of-the-art language models keep track of really, really long linguistic contexts. Humans don’t do that,” she says.
Chatbots can retain long strings of dialogue, using those words to tailor their responses as a conversation progresses, she explains. Humans, on the other hand, must cope with a more limited memory. While we can keep track of information as it is conveyed, we only store a string of about eight words as we listen or read. “We get linguistic input, we crunch it up, we extract some kind of meaning representation, presumably in some more abstract format, and then we discard the exact linguistic stream because we don’t need it anymore,” Fedorenko explains.
Language models aren’t able to fill in gaps in conversation with their own knowledge and awareness in the same way a person can, Ivanova adds. “That’s why so far they have to keep track of every single input word,” she says. “If we want a model that models specifically the [human] language network, we don’t need to have this large context window. It would be very cool to train those models on those short windows of context and see if it’s more similar to the language network.”
Multimodal intelligence
Despite these parallels, Fedorenko’s lab has also shown that there are plenty of things language circuits do not do. The brain calls on other circuits to solve math problems, write computer code, and carry out myriad other cognitive processes. Their work makes it clear that in the brain, language and thought are not the same.
That’s borne out by what cognitive neuroscientists like Kanwisher have learned about the functional organization of the human brain, where circuit components are dedicated to surprisingly specific tasks, from language processing to face recognition.
“The upshot of cognitive neuroscience over the last 25 years is that the human brain really has quite a degree of modular organization,” Kanwisher says. “You can look at the brain and say, ‘what does it tell us about the nature of intelligence?’ Well, intelligence is made up of a whole bunch of things.”
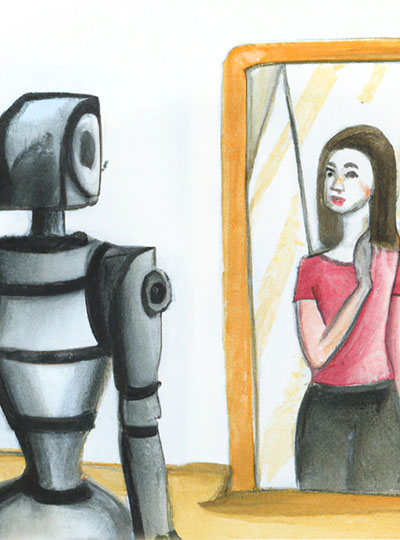
In January, Fedorenko, Kanwisher, Ivanova, and colleagues shared an extensive analysis of the capabilities of large language models. After assessing models’ performance on various language-related tasks, they found that despite their mastery of linguistic rules and patterns, such models don’t do a good job using language in real-world situations. From a neuroscience perspective, that kind of functional competence is distinct from formal language competence, calling on not just language-processing circuits but also parts of the brain that store knowledge of the world, reason, and interpret social interactions.
Language is a powerful tool for understanding the world, they say, but it has limits.
“If you train on language prediction alone, you can learn to mimic certain aspects of thinking,” Ivanova says. “But it’s not enough. You need a multimodal system to carry out truly intelligent behavior.”
The team concluded that while AI language models do a very good job using language, they are incomplete models of human thought. For machines to truly think like humans, Ivanova says, they will need a combination of different neural nets all working together, in the same way different networks in the human brain work together to achieve complex cognitive tasks in the real world.
It remains to be seen whether such models would excel in the tech world, but they could prove valuable for revealing insights into human cognition — perhaps in ways that will inform engineers as they strive to build systems that better replicate human intelligence.