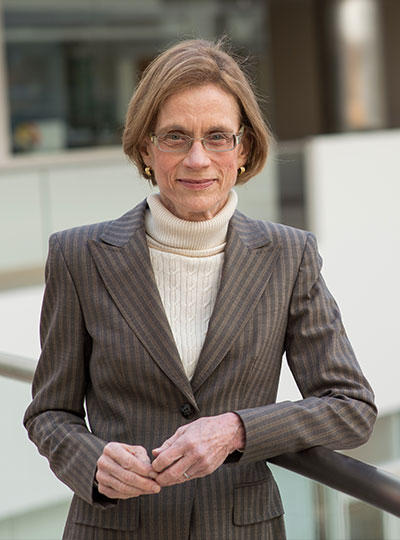
Dopamine is a powerful signal in the brain, influencing our moods, motivations, movements, and more. The neurotransmitter is crucial for reward-based learning, a function that may be disrupted in a number of psychiatric conditions, from mood disorders to addiction. Now, researchers led by Ann Graybiel, an investigator at MIT’s McGovern Institute, have found surprising patterns of dopamine signaling that suggest neuroscientists may need to refine their model of how reinforcement learning occurs in the brain. The team’s findings were published October 14, 2024, in the journal Nature Communications.
Dopamine plays a critical role in teaching people and other animals about the cues and behaviors that portend both positive and negative outcomes; the classic example of this type of learning is the dog that Ivan Pavlov trained to anticipate food at the sound of bell. Graybiel explains that according to the standard model of reinforcement learning, when an animal is exposed to a cue paired with a reward, dopamine-producing cells initially fire in response to the reward. As animals learn the association between the cue and the reward, the timing of dopamine release shifts, so it becomes associated with the cue instead of the reward itself.
But with new tools enabling more detailed analyses of when and where dopamine is released in the brain, Graybiel’s team is finding that this model doesn’t completely hold up. The group started picking up clues that the field’s model of reinforcement learning was incomplete more than ten years ago, when Mark Howe, a graduate student in the lab, noticed that the dopamine signals associated with reward were released not in a sudden burst the moment a reward was obtained, but instead before that, building gradually as a rat got closer to its treat. Dopamine might actually be communicating to the rest of the brain the proximity of the reward, they reasoned. “That didn’t fit at all with the standard, canonical model,” Graybiel says.
Dopamine dynamics
As other neuroscientists considered how a model of reinforcement learning could take those findings into account, Graybiel and postdoctoral researcher Min Jung Kim decided it was time to take a closer look at dopamine dynamics.
“We thought, let’s go back to the most basic kind of experiment and start all over again,” Graybiel says.
That meant using sensitive new dopamine sensors to track the neurotransmitter’s release in the brains of mice as they learned to associated a blue light with a satisfying sip of water. The team focused its attention on the striatum, a region within the brain’s basal ganglia, where neurons use dopamine to influence neural circuits involved in a variety of processes, including reward-based learning.
The researchers found that the timing of dopamine release varied in different parts of the striatum. But nowhere did Graybiel’s team find a transition in dopamine release timing from the time of the reward to the time to the cue—the key transition predicted by the standard model of reinforcement learning model.
In the team’s simplest experiments, where every time a mouse saw a light it was paired with a reward, the lateral part of the striatum reliably released dopamine when animals were given their water. This strong response to the reward never diminished, even as the mice learned to expect the reward when they saw a light. In the medial part of the striatum, in contrast, dopamine was never released at the time of the reward. Cells there always fired when a mouse saw the light, even early in the learning process. This was puzzling, Graybiel says, because at the beginning of learning, dopamine would have been predicted to respond to the reward itself.
The patterns of dopamine release became even more unexpected when Graybiel’s team introduced a second light into its experimental setup. The new light, in a different position than the first, did not signal a reward. Mice watched as either light was given as the cue, one at a time, with water accompanying only the original cue.
In these experiments, when the mice saw the reward-associated light, dopamine release went up in the centromedial striatum and surprisingly, stayed up until the reward was delivered. In the lateral part of the region, dopamine also involved a sustained period where signaling plateaued.
Graybiel says she was surprised to see how much dopamine responses changed when the experimenters introduce the second light. The responses to the rewarded light were different when the other light could be shown in other trials, even though the mice saw only one light at a time. “There must be a cognitive aspect to this that comes into play,” she says. “The brain wants to hold onto the information that the cue has come on for a while.” Cells in the striatum seem to achieve this through the sustained dopamine release that continued during the brief delay between the light and the reward in the team’s experiments. Indeed, Graybiel said, while this kind of sustained dopamine release has not previously been linked to reinforcement learning, it is reminiscent of sustained signaling that has been tied to working memory in other parts of the brain.
Reinforcement learning, reconsidered
Ultimately, Graybiel says, “many of our results didn’t fit reinforcement learning models as traditionally—and by now canonically—considered.” That suggests neuroscientists’ understanding of this process will need to evolve as part of the field’s deepening understanding of the brain. “But this is just one step to help us all refine our understanding and to have reformulations of the models of how basal ganglia influence movement and thought and emotion. These reformulations will have to include surprises about the reinforcement learning system vis-á-vis these plateaus, but they could possibly give us insight into how a single experience can linger in this reinforcement-related part of our brains,” she says.
This study was funded by the National Institutes of Health, the William N. & Bernice E. Bumpus Foundation, the Saks Kavanaugh Foundation, the CHDI Foundation, Joan and Jim Schattinger, and Lisa Yang.