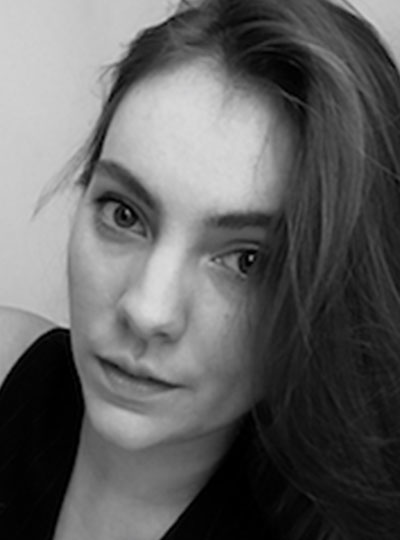
Computational neuroscientist Sarah Schwettmann, who works in the Center for Brains, Minds, and Machines at the McGovern Institute, is one of three instructors behind the cross-disciplinary course 9.S52/9.S916 (Vision in Art and Neuroscience), which introduces students to core concepts in visual perception through the lenses of art and neuroscience.
Supported by a faculty grant from the Center for Art, Science and Technology at MIT (CAST) for the past two years, the class is led by Pawan Sinha, a professor of vision and computational neuroscience in the Department of Brain and Cognitive Sciences. They are joined in the course by Seth Riskin SM ’89, a light artist and the manager of the MIT Museum Studio and Compton Gallery, where the course is taught. Schwettman discussed the combination of art and science in an educational setting.
Q: How have the three of you approached this cross-disciplinary class in art and neuroscience?
A: Discussions around this intersection often consider what each field has to offer the other. We take a different approach, one I refer to as occupying the gap, or positioning ourselves between the two fields and asking what essential questions underlie them both. One question addresses the nature of the human relationship to the world. The course suggests one answer: This relationship is fundamentally creative, from the brain’s interpretation of incoming sensory data in perception, to the explicit construction of experiential worlds in art.
Neuroscience and art, therefore, each provide a set of tools for investigating different levels of the constructive process. Through neuroscience, we develop a specific understanding of the models of the world that the brain uses to make sense of incoming visual data. With articulation of those models, we can engineer types of inputs that interact with visual processing architecture in particularly exquisite ways, and do so reliably, giving artists a toolkit for remixing and modulating experience. In the studio component of the course, we experiment with this toolkit and collectively move it forward.
While designing the course, Pawan, Seth, and I found that we were each addressing a similar set of questions, the same that motivate the class, through our own research and practice. In parallel to computational vision research, Professor Sinha leads a humanitarian initiative called Project Prakash, which provides treatment to blind children in India and explores the development of vision following the restoration of sight. Where does structure in perception originate? As an artist in the MIT Museum Studio, Seth works with articulated light to sculpt structured visual worlds out of darkness. I also live on this interface where the brain meets the world — my research in the Department of Brain and Cognitive Sciences examines the neural basis of mental models for simulating physics. Linking our work in the course is an experiment in synthesis.
Q: What current research in vision, neuroscience, and art are being explored at MIT, and how does the class connect it to hands-on practice?
A: Our brains build a rich world of experience and expectation from limited and noisy sensory data with infinite potential interpretations. In perception research, we seek to discover how the brain finds more meaning in incoming data than is explained by the signal alone. Work being done at MIT around generative models addresses this, for instance in the labs of Josh Tenenbaum and Josh McDermott in the Department of Brain and Cognitive Sciences. Researchers present an ambiguous visual or auditory stimulus and by probing someone’s perceptual interpretation, they get a handle on the structures that the mind generates to interpret incoming data, and they can begin to build computational models of the process.
In Vision in Art and Neuroscience, we focus on the experiential as well as the experimental, probing the perceiver’s experience of structure-generating process—perceiving perception itself.
As instructors, we face the pedagogical question: what exercises, in the studio, can evoke so striking an experience of students’ own perception that cutting edge research takes on new meaning, understood in the immediacy of seeing? Later in the semester, students face a similar question as artists: How can one create visual environments where viewers experience their own perceptual processing at work? Done well, this experience becomes the artwork itself. Early in the course, students explore the Ganzfeld effect, popularized by artist James Turrell, where the viewer is exposed to an unstructured visual field of uniform illumination. In this experience, one feels the mind struggling to fit models of the world to unstructured input, and attempting this over and over again — an interpretation process which often goes unnoticed when input structure is expected by visual processing architecture. The progression of the course modules follows the hierarchy of visual processing in the brain, which builds increasingly complex interpretations of visual inputs, from brightness and edges to depth, color, and recognizable form.
MIT students first encounter those concepts in the seminar component of the course at the beginning of each week. Later in the week, students translate findings into experimental approaches in the studio. We work with light directly, from introducing a single pinpoint of light into an otherwise completely dark room, to building intricate environments using programmable electronics. Students begin to take this work into their own hands, in small groups and individually, culminating in final projects for exhibition. These exhibitions are truly a highlight of the course. They’re often one of the first times that students have built and shown artworks. That’s been a gift to share with the broader MIT community, and a great learning experience for students and instructors alike.
Q: How has that approach been received by the MIT community?
A: What we’re doing has resonated across disciplines: In addition to neuroscience, we have students and researchers joining us from computer science, mechanical engineering, mathematics, the Media Lab, and ACT (the Program in Art, Culture, and Technology). The course is growing into something larger, a community of practice interested in applying the scientific methodology we develop to study the world, to probe experience, and to articulate models for its generation and replication.
With a mix of undergraduates, graduates, faculty, and artists, we’ve put together installations and symposia — including three on campus so far. The first of these, “Perceiving Perception,” also led to a weekly open studio night where students and collaborators convene for project work. Our second exhibition, “Dessert of the Real,” is on display this spring in the Compton Gallery. This April we’re organizing a symposium in the studio featuring neuroscientists, computer scientists, artists and researchers from MIT and Harvard. We’re reaching beyond campus as well, through off-site installations, collaborations with museums — including the Metropolitan Museum of Art and the Peabody Essex Museum — and a partnership with the ZERO Group in Germany.
We’re eager to involve a broad network of collaborators. It’s an exciting moment in the fields of neuroscience and computing; there is great energy to build technologies that perceive the world like humans do. We stress on the first day of class that perception is a fundamentally creative act. We see the potential for models of perception to themselves be tools for scaling and translating creativity across domains, and for building a deeply creative relationship to our environment.