In the hit T.V. show “Westworld,” Dolores Abernathy, a golden-tressed belle, lives in the days when Manifest Destiny still echoed in America. She begins to notice unusual stirrings shaking up her quaint western town—and soon discovers that her skin is synthetic, and her mind, metal. She’s a cyborg meant to entertain humans. The key to her autonomy lies in reaching consciousness.
Shows like “Westworld” and other media probe the idea of consciousness, attempting to nail down a definition of the concept. However, though humans have ruminated on consciousness for centuries, we still don’t have a solid definition (even the Merriam-Webster dictionary lists five). One framework suggests that consciousness is any experience, from eating a candy bar to heartbreak. Another argues that it is how certain stimuli influence one’s behavior.
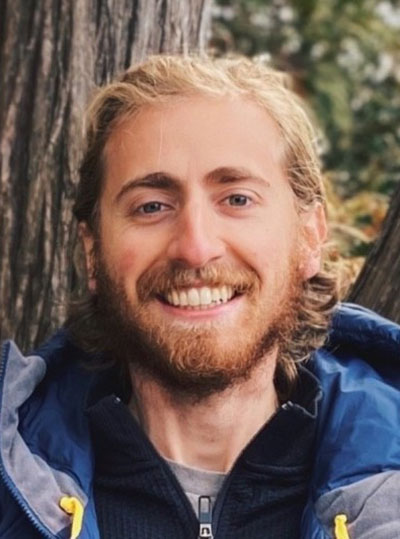
While some search for a philosophical explanation, MIT graduate student Adam Eisen seeks a scientific one.
Eisen studies consciousness in the lab of Ila Fiete, an associate investigator at the McGovern Institute. His work melds seemingly opposite fields, using mathematical models to quantitatively explain, and thereby ground, the loftiness of consciousness.
In the Fiete lab, Eisen leverages computational methods to compare the brain’s electrical signals in an awake, conscious state to those in an unconscious state via anesthesia—which dampens communication between neurons so people feel no pain or become unconscious.
“What’s nice about anesthesia is that we have a reliable way of turning off consciousness,” says Eisen.
“So we’re now able to ask: What’s the fluctuation of electrical activity in a conscious versus unconscious brain? By characterizing how these states vary—with the precision enabled by computational models—we can start to build a better intuition for what underlies consciousness.”
Theories of consciousness
How are scientists thinking about consciousness? Eisen says that there are four major theories circulating in the neuroscience sphere. These theories are outlined below.
Global workspace theory
Consider the placement of your tongue in your mouth. This sensory information is always there, but you only notice the sensation when you make the effort to think about it. How does this happen?
“Global workspace theory seeks to explain how information becomes available to our consciousness,” he says. “This is called access consciousness—the kind that stores information in your mind and makes it available for verbal report. In this view, sensory information is broadcasted to higher-level regions of the brain by a process called ignition.” The theory proposes that widespread jolts of neuronal activity or “spiking” are essential for ignition, like how a few claps can lead to an audience applause. It’s through ignition that we reach consciousness.
Eisen’s research in anesthesia suggests, though, that not just any spiking will do. There needs to be a balance: enough activity to spark ignition, but also enough stability such that the brain doesn’t lose its ability to respond to inputs and produce reliable computations to reach consciousness.
Higher order theories
Let’s say you’re listening to “Here Comes The Sun” by The Beatles. Your brain processes the medley of auditory stimuli; you hear the bouncy guitar, upbeat drums, and George Harrison’s perky vocals. You’re having a musical experience—what it’s like to listen to music. According to higher-order theories, such an experience unlocks consciousness.
“Higher-order theories posit that a conscious mental state involves having higher-order mental representations of stimuli—usually in the higher levels of the brain responsible for cognition—to experience the world,” Eisen says.
Integrated information theory
“Imagine jumping into a lake on a warm summer day. All components of that experience—the feeling of the sun on your skin and the coolness of the water as you submerge—come together to form your ‘phenomenal consciousness,’” Eisen says. If the day was slightly less sunny or the water a fraction warmer, he explains, the experience would be different.
“Integrated information theory suggests that phenomenal consciousness involves an experience that is irreducible, meaning that none of the components of that experience can be separated or altered without changing the experience itself,” he says.
Attention schema theory
Attention schema theory, Eisen explains, says ‘attention’ is the information that we are focused on in the world, while ‘awareness’ is the model we have of our attention. He cites an interesting psychology study to disentangle attention and awareness.
In the study, the researchers showed human subjects a mixed sequence of two numbers and six letters on a computer. The participants were asked to report back what the numbers were. While they were doing this task, faintly detectable dots moved across the screen in the background. The interesting part, Eisen notes, is that people weren’t aware of the dots—that is, they didn’t report that they saw them. But despite saying they didn’t see the dots, people performed worse on the task when the dots were present.
“This suggests that some of the subjects’ attention was allocated towards the dots, limiting their available attention for the actual task,” he says. “In this case, people’s awareness didn’t track their attention. The subjects were not aware of the dots, even though the study shows that the dots did indeed affect their attention.”
The science behind consciousness
Eisen notes that a solid understanding of the neural basis of consciousness has yet to be cemented. However, he and his research team are advancing in this quest. “In our work, we found that brain activity is more ‘unstable’ under anesthesia, meaning that it lacks the ability to recover from disturbances—like distractions or random fluctuations in activity—and regain a normal state,” he says.
He and his fellow researchers believe this is because the unconscious brain can’t reliably engage in computations like the conscious brain does, and sensory information gets lost in the noise. This crucial finding points to how the brain’s stability may be a cornerstone of consciousness.
There’s still more work to do, Eisen says. But eventually, he hopes that this research can help crack the enduring mystery of how consciousness shapes human existence. “There is so much complexity and depth to human experience, emotion, and thought. Through rigorous research, we may one day reveal the machinery that gives us our common humanity.”