Some people, especially those in public service, perform admirable feats—healthcare workers fighting to keep patients alive or a first responder arriving at the scene of a car crash. But the emotional weight can become a mental burden. Research has shown that emergency personnel are at elevated risk for mental health challenges like post-traumatic stress disorder. How can people undergo such stressful experiences and also maintain their well-being?
A new study from the McGovern Institute reveals that a cognitive strategy focused on social good may be effective in helping people cope with distressing events. The research team found that the approach was comparable to another well-established emotion regulation strategy, unlocking a new tool for dealing with highly adverse situations.
“How you think can improve how you feel.”
– John Gabrieli
“This research suggests that the social good approach might be particularly useful in improving well-being for those constantly exposed to emotionally taxing events,” says John Gabrieli, the Grover Hermann Professor of Health Sciences and Technology and a professor of brain and cognitive sciences at MIT, who is a senior author of the paper.
The study, published today in PLOS ONE, is the first to examine the efficacy of this cognitive strategy. Nancy Tsai, a postdoctoral research scientist in Gabrieli’s lab at the McGovern Institute, is the lead author of the paper.
Emotion regulation tools
Emotion regulation is the ability to mentally reframe how we experience emotions—a skill critical to maintaining good mental health. Doing so can make one feel better when dealing with adverse events, and emotion regulation has been shown to boost emotional, social, cognitive, and physiological outcomes across the lifespan.
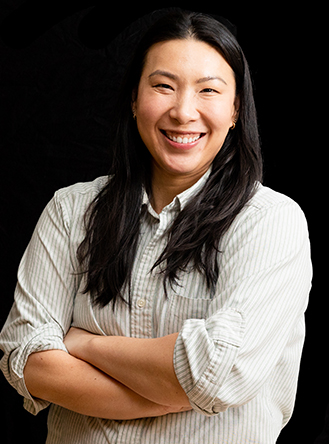
One emotion regulation strategy is “distancing,” where a person copes with a negative event by imagining it as happening far away, a long time ago, or from a third-person perspective. Distancing has been well-documented as a useful cognitive tool, but it may be less effective in certain situations, especially ones that are socially charged—like a firefighter rescuing a family from a burning home. Rather than distancing themselves, a person may instead be forced to engage directly with the situation.
“In these cases, the ‘social good’ approach may be a powerful alternative,” says Tsai. “When a person uses the social good method, they view a negative situation as an opportunity to help others or prevent further harm.” For example, a firefighter experiencing emotional distress might focus on the fact that their work enables them to save lives. The idea had yet to be backed by scientific investigation, so Tsai and her team, alongside Gabrieli, saw an opportunity to rigorously probe this strategy.
A novel study
The MIT researchers recruited a cohort of adults and had them complete a questionnaire to gather information including demographics, personality traits, and current well-being, as well as how they regulated their emotions and dealt with stress. The cohort was randomly split into two groups: a distancing group and a social good group. In the online study, each group was shown a series of images that were either neutral (such as fruit) or contained highly aversive content (such as bodily injury). Participants were fully informed of the types of images they might see and could opt out of the study at any time.
Each group was asked to use their assigned cognitive strategy to respond to half of the negative images. For example, while looking at a distressing image, a person in the distancing group could have imagined that it was a screenshot from a movie. Conversely, a subject in the social good group might have responded to the image by envisioning that they were a first responder saving people from harm. For the other half of the negative images, participants were asked to only look at them and pay close attention to their emotions. The researchers asked the participants how they felt after each image was shown.
Social good as a potent strategy
The MIT team found that distancing and social good approaches helped diminish negative emotions. Participants reported feeling better when they used these strategies after viewing adverse content compared to when they did not and stated that both strategies were easy to implement.
The results also revealed that, overall, distancing yielded a stronger effect. Importantly, however, Tsai and Gabrieli believe that this study offers compelling evidence for social good as a powerful method better suited to situations when people cannot distance themselves, like rescuing someone from a car crash, “Which is more probable for people in the real world,” notes Tsai. Moreover, the team discovered that people who most successfully used the social good approach were more likely to view stress as enhancing rather than debilitating. Tsai says this link may point to psychological mechanisms that underlie both emotion regulation and how people respond to stress.
“The social good approach may be a potent strategy to combat the immense emotional demands of certain professions.”
– John Gabrieli
Additionally, the results showed that older adults used the cognitive strategies more effectively than younger adults. The team suspects that this is probably because, as prior research has shown, older adults are more adept at regulating their emotions likely due to having greater life experiences. The authors note that successful emotion regulation also requires cognitive flexibility, or having a malleable mindset to adapt well to different situations.
“This is not to say that people, such as physicians, should reframe their emotions to the point where they fully detach themselves from negative situations,” says Gabrieli. “But our study shows that the social good approach may be a potent strategy to combat the immense emotional demands of certain professions.”
The MIT team says that future studies are needed to further validate this work, and that such research is promising in that it can uncover new cognitive tools to equip individuals to take care of themselves as they bravely assume the challenge of taking care of others.