By studying how primates mentally measure time, scientists at MIT’s McGovern Institute have discovered that the brain runs an internal clock whose speed is set by prior experience. In new experiences, the brain closely tracks how elapsed time intervals differ from its preset expectation—indicating that for the brain, time is relative.
The findings, reported September 15, 2021, in the journal Neuron, help explain how the brain uses past experience to make predictions—a powerful strategy for navigating a complex and ever-changing world. The research was led by McGovern Investigator Mehrdad Jazayeri, who is working to understand how the brain forms internal models of the world.
Internal clock
Sensory information tells us a lot about our environment, but the brain needs more than data, Jazayeri says. Internal models are vital for understanding the relationships between things, making generalizations, and interpreting and acting on our perceptions. They help us focus on what’s most important and make predictions about our surroundings, as well as the consequences of our actions. “To be efficient in learning about the world and interacting with the world, we need those predictions,” Jazayeri says. When we enter a new grocery store, for example, we don’t have to check every aisle for the peanut butter, because we know it is likely to be near the jam. Likewise, an experienced racquetball player knows how the ball will move when her paddle hits it a certain way.
Jazayeri’s team was interested in how the brain might make predictions about time. Previously, his team showed how neurons in the frontal cortex—a part of the brain involved in planning—can tick off the passage of time like a metronome. By training monkeys to use an eye movement to indicate the duration of time that separated two flashes of light, they found that cells that track time during this task cooperate to form an adjustable internal clock. Those cells generate a pattern of activity that can be drawn out to measure long time intervals or compressed to track shorter ones. The changes in these signal dynamics reflect elapsed time so precisely that by monitoring the right neurons, Jazayeri’s team can determine exactly how fast a monkey’s internal clock is running.
Predictive processing
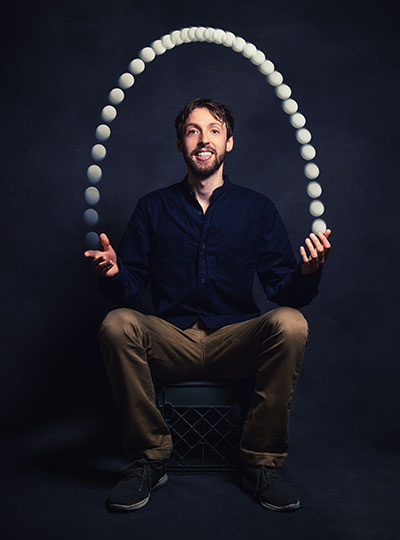
For their most recent experiments, graduate student Nicolas Meirhaeghe designed a series of experiments in which the delay between the two flashes of light changed as the monkeys repeated the task. Sometimes the flashes were separated by just a fraction of a second, sometimes the delay was a bit longer. He found that the time-keeping activity pattern in the frontal cortex occurred over different time scales as the monkeys came to expect delays of different durations. As the duration of the delay fluctuated, the brain appeared to take all prior experience into account, setting the clock to measure the average of those times in anticipation of the next interval.
The behavior of the neurons told the researchers that as a monkey waited for a new set of light cues, it already had an expectation about how long the delay would be. To make such a prediction, Meirhaeghe says, “the brain has no choice but to use all the different values that you perceive from your experience, average those out, and use this as the expectation.”
By analyzing neuronal behavior during their experiments, Jazayeri and Meirhaeghe determined that the brain’s signals were not encoding the full time elapsed between light cues, but instead how that time differed from the predicted time. Calculating this prediction error enabled the monkeys to report back how much time had elapsed.
Neuroscientists have suspected that this strategy, known as predictive processing, is widely used by the brain—although until now there has been little evidence of it outside early sensory areas. “You have a lot of stimuli that are coming from the environment, but lots of stimuli are actually predictable,” Meirhaeghe says. “The idea is that your brain is learning through experience patterns in the environment, and is subtracting your expectation from the incoming signal. What the brain actually processes in the end is the result of this subtraction.”
Finally, the researchers investigated the brain’s ability to update its expectations about time. After presenting monkeys with delays within a particular time range, they switched without warning to times that fluctuated within a new range. The brain responded quickly, updating its internal clock. “If you look inside the brain, after about 100 trials the monkeys have already figured out that these statistics have changed,” says Jazayeri.
It took longer, however—as many as 1,000 trials—for the monkeys to change their behavior in response to the change. “It seems like this prediction, and updating the internal model about the statistics of the world, is way faster than our muscles are able to implement,” Jazayeri says. “Our motor system is kind of lagging behind what our cognitive abilities tell us.” This makes sense, he says, because not every change in the environment merits a change in behavior. “You don’t want to be distracted by every small thing that deviates from your prediction. You want to pay attention to things that have a certain level of consistency.”