Our brains are incredibly good at processing faces, and even have specific regions specialized for this function. But what face dimensions are we observing? Do we observe general properties first, then look at the details? Or are dimensions such as gender or other identity details decoded interdependently? In a study published today in Nature Communications, the Kanwisher lab measured the response of the brain to faces in real time, and found that the brain first decodes properties such as gender and age before drilling down to the specific identity of the face itself.
While functional magnetic resonance imaging (fMRI) has revealed an incredible level of detail about which regions of the brain respond to faces, the technology is less effective at telling us when these brain regions become activated. This is because fMRI measures brain activity by detecting changes in blood flow; when neurons become active, local blood flow to those brain regions increases. However, fMRI works too slowly to keep up with the brain’s millisecond-by-millisecond dynamics. Enter magnetoencephalography (MEG), a technique developed by MIT physicist David Cohen that detects the minuscule fluctuations in magnetic field that occur with the electrical activity of neurons. This allows better temporal resolution of neural activity.
McGovern Investigator Nancy Kanwisher and postdoc Katharina Dobs, along with their co-authors Leyla Isik and Dimitrios Pantazis, selected this temporally precise approach to measure the time it takes for the brain to respond to different dimensional features of faces.
“From a brief glimpse of a face, we quickly extract all this rich multidimensional information about a person, such as their sex, age, and identity,” explains Dobs. “I wanted to understand how the brain accomplishes this impressive feat, and what the neural mechanisms are that underlie this effect, but no one had measured the time scales of responses to these features in the same study.”
Previous studies have shown that people with prosopagnosia, a condition characterized by the inability to identify familiar faces, have no trouble determining gender, suggesting these features may be independent. “But examining when the brain recognizes gender and identity, and whether these are interdependent features is less clear,” explains Dobs.
By recording the brain activity of subjects in the MEG, Dobs and her co-authors found that the brain responds to coarse features, such as the gender of a face, much faster than the identity of the face itself. Their data showed that, in as little as 60-70 milliseconds, the brain begins to decode the age and gender of a person. Roughly 30 milliseconds later — at around 90 milliseconds — the brain begins processing the identity of the face.
After establishing a paradigm for measuring responses to these face dimensions, the authors then decided to test the effect of familiarity. It’s generally understood that the brain processes information about “familiar faces” more robustly than unfamiliar faces. For example, our brains are adept at recognizing actress Scarlett Johansson across multiple photographs, even if her hairstyle is different in each picture. Our brains have a much harder time, however, recognizing two images of the same person if the face is unfamiliar.
“Actually, for unfamiliar faces the brain is easily fooled,” Dobs explains, “variations in images, shadows, changes in hair color or style, quickly lead us to think we are looking at a different person. Conversely, we have no problem if a familiar face is in shadow, or a friend changes their hair style. But we didn’t know why familiar face perception is much more robust, whether this is due to better feed forward processing, or based on later memory retrieval.”
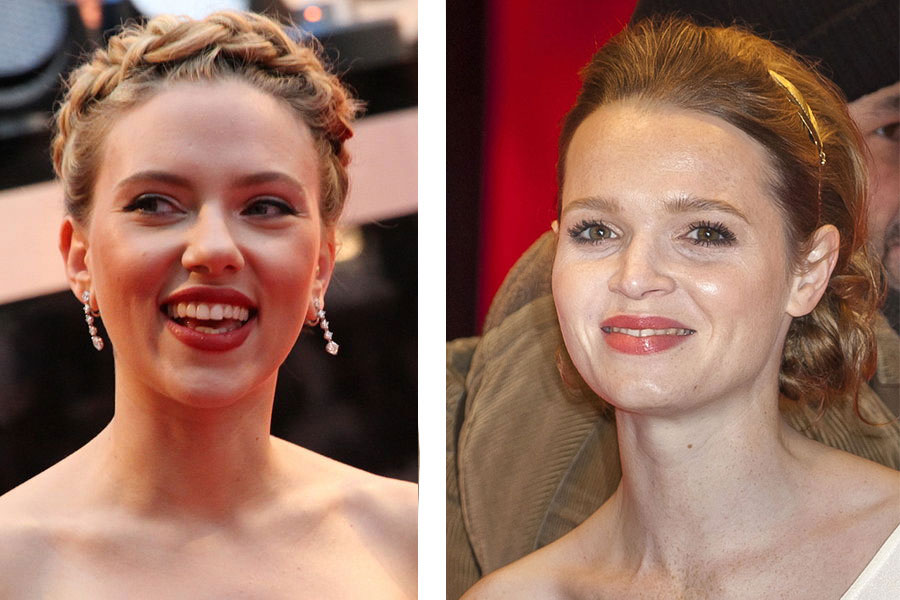
To test the effect of familiarity, the authors measured brain responses while the subjects viewed familiar faces (American celebrities) and unfamiliar faces (German celebrities) in the MEG. Surprisingly, they found that subjects recognize gender more quickly in familiar faces than unfamiliar faces. For example our brains decode that actor Scarlett Johansson is female, before we even realize she is Scarlett Johansson. And for the less familiar German actor, Karoline Herfurth, our brains unpack the same information less well.
Dobs and co-authors argue that better gender and identity recognition is not “top-down” for familiar faces, meaning that improved responses to familiar faces is not about retrieval of information from memory, but rather, a feed-forward mechanism. They found that the brain responds to facial familiarity at a much slower time scale (400 milliseconds) than it responds to gender, suggesting that the brain may be remembering associations related to the face (Johansson = Lost in Translation movie) in that longer timeframe.
This is good news for artificial intelligence. “We are interested in whether feed-forward deep learning systems can learn faces using similar mechanisms,” explains Dobs, “and help us to understand how the brain can process faces it has seen before in the absence of pulling on memory.”
When it comes to immediate next steps, Dobs would like to explore where in the brain these facial dimensions are extracted, how prior experience affects the general processing of objects, and whether computational models of face processing can capture these complex human characteristics.