Algorithms of intelligence
How the DiCarlo lab fine-tuned its machine vision system to precisely control neural activity.
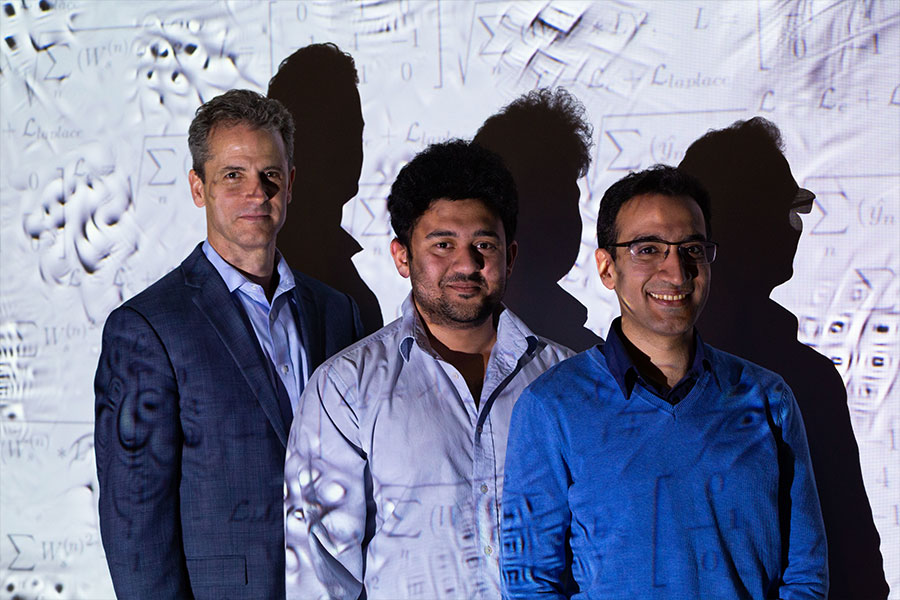
The following post is adapted from a story featured in a recent Brain Scan newsletter.
Machine vision systems are more and more common in everyday life, from social media to self-driving cars, but training artificial neural networks to “see” the world as we do—distinguishing cyclists from signposts—remains challenging. Will artificial neural networks ever decode the world as exquisitely as humans? Can we refine these models and influence perception in a person’s brain just by activating individual, selected neurons? The DiCarlo lab, including CBMM postdocs Kohitij Kar and Pouya Bashivan, are finding that we are surprisingly close to answering “yes” to such questions, all in the context of accelerated insights into artificial intelligence at the McGovern Institute for Brain Research, CBMM, and the Quest for Intelligence at MIT.
Precision Modeling
Beyond light hitting the retina, the recognition process that unfolds in the visual cortex is key to truly “seeing” the surrounding world. Information is decoded through the ventral visual stream, cortical brain regions that progressively build a more accurate, fine-grained, and accessible representation of the objects around us. Artificial neural networks have been modeled on these elegant cortical systems, and the most successful models, deep convolutional neural networks (DCNNs), can now decode objects at levels comparable to the primate brain. However, even leading DCNNs have problems with certain challenging images, presumably due to shadows, clutter, and other visual noise. While there’s no simple feature that unites all challenging images, the quest is on to tackle such images to attain precise recognition at a level commensurate with human object recognition.
“One next step is to couple this new precision tool with our emerging understanding of how neural patterns underlie object perception. This might allow us to create arrangements of pixels that look nothing like, for example, a cat, but that can fool the brain into thinking it’s seeing a cat.”- James DiCarlo
In a recent push, Kar and DiCarlo demonstrated that adding feedback connections, currently missing in most DCNNs, allows the system to better recognize objects in challenging situations, even those where a human can’t articulate why recognition is an issue for feedforward DCNNs. They also found that this recurrent circuit seems critical to primate success rates in performing this task. This is incredibly important for systems like self-driving cars, where the stakes for artificial visual systems are high, and faithful recognition is a must.
Now you see it
As artificial object recognition systems have become more precise in predicting neural activity, the DiCarlo lab wondered what such precision might allow: could they use their system to not only predict, but to control specific neuronal activity?
To demonstrate the power of their models, Bashivan, Kar, and colleagues zeroed in on targeted neurons in the brain. In a paper published in Science, they used an artificial neural network to generate a random-looking group of pixels that, when shown to an animal, activated the team’s target, a target they called “one hot neuron.” In other words, they showed the brain a synthetic pattern, and the pixels in the pattern precisely activated targeted neurons while other neurons remained relatively silent.
These findings show how the knowledge in today’s artificial neural network models might one day be used to noninvasively influence brain states with neural resolution. Such precise systems would be useful as we look to the future, toward visual prosthetics for the blind. Such a precise model of the ventral visual stream would have been incon-ceivable not so long ago, and all eyes are on where McGovern researchers will take these technologies in the coming years.