A new study from the McGovern Institute shows how interests can modulate language processing in children’s brains and paves the way for personalized brain research.
The paper, which appears in Imaging Neuroscience, was conducted in the lab of McGovern Institute Investigator John Gabrieli, and led by senior author Anila D’Mello, a former McGovern postdoctoral fellow and current assistant professor at the University of Texas Southwestern Medical Center and the University of Texas at Dallas.
“Traditional studies give subjects identical stimuli to avoid confounding the results,” says Gabrieli, who is also the Grover Hermann Professor of Health Sciences and Technology and a professor of brain and cognitive sciences at MIT.
“However, our research tailored stimuli to each child’s interest, eliciting stronger—and more consistent—activity patterns in the brain’s language regions across individuals.” – John Gabrieli
Funded by the Hock E. Tan and K. Lisa Yang Center for Autism Research in MIT’s Yang Tan Collective, this work unveils a new paradigm that challenges current methods and shows how personalization can be a powerful strategy in neuroscience. The paper’s co-first authors are Halie Olson, a postdoctoral associate at the McGovern Institute, and Kristina Johnson, an assistant professor at Northeastern University and former doctoral student at the MIT Media Lab. “Our research integrates participants’ lived experiences into the study design,” says Johnson. “This approach not only enhances the validity of our findings but also captures the diversity of individual perspectives, often overlooked in traditional research.”
Taking interest into account
When it comes to language, our interests are like operators behind the switchboard. They guide what we talk about and who we talk to. Research suggests that interests are also potent motivators and can help improve language skills. For instance, children score higher on reading tests when the material covers topics that are interesting to them.
But neuroscience has shied away from using personal interests to study the brain, especially in the realm of language. This is mainly because interests, which vary between people, could throw a wrench into experimental control—a core principle that drives scientists to limit factors that can muddle the results.
Gabrieli, D’Mello, Olson, and Johnson ventured into this unexplored territory. The team wondered if tailoring language stimuli to children’s interests might lead to higher responses in language regions of the brain. “Our study is unique in its approach to control the kind of brain activity our experiments yield, rather than control the stimuli we give subjects,” says D’Mello. “This stands in stark contrast to most neuroimaging studies that control the stimuli but might introduce differences in each subject’s level of interest in the material.”
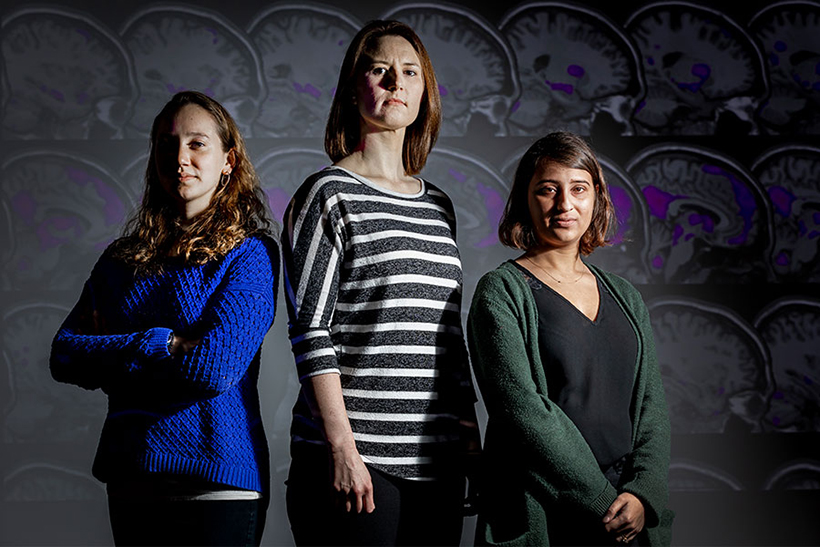
In their recent study, the authors recruited a cohort of 20 children to investigate how personal interests affected the way the brain processes language. Caregivers described their child’s interests to the researchers, spanning baseball, train lines, Minecraft, and musicals. During the study, children listened to audio stories tuned to their unique interests. They were also presented with audio stories about nature (this was not an interest among the children) for comparison. To capture brain activity patterns, the team used functional magnetic resonance imaging (fMRI), which measures changes in blood flow caused by underlying neural activity.
New insights into the brain
“We found that, when children listened to stories about topics they were really interested in, they showed stronger neural responses in language areas than when they listened to generic stories that weren’t tailored to their interests,” says Olson. “Not only does this tell us how interests affect the brain, but it also shows that personalizing our experimental stimuli can have a profound impact on neuroimaging results.”
The researchers noticed a particularly striking result. “Even though the children listened to completely different stories, their brain activation patterns were more overlapping with their peers when they listened to idiosyncratic stories compared to when they listened to the same generic stories about nature,” says D’Mello. This, she notes, points to how interests can boost both the magnitude and consistency of signals in language regions across subjects without changing how these areas communicate with each other.
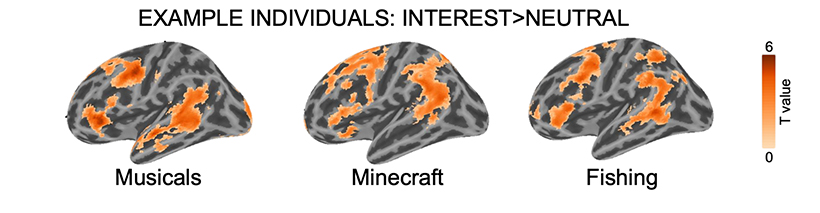
Gabrieli noted another finding: “In addition to the stronger engagement of language regions for content of interest, there was also stronger activation in brain regions associated with reward and also with self-reflection.” Personal interests are individually relevant and can be rewarding, potentially driving higher activation in these regions during personalized stories.
These personalized paradigms might be particularly well-suited to studies of the brain in unique or neurodivergent populations. Indeed, the team is already applying these methods to study language in the brains of autistic children.
This study breaks new ground in neuroscience and serves as a prototype for future work that personalizes research to unearth further knowledge of the brain. In doing so, scientists can compile a more complete understanding of the type of information that is processed by specific brain circuits and more fully grasp complex functions such as language.