This story also appears in the Winter 2025 issue of BrainScan.
___
McGovern investigator Nancy Kanwisher and her team have big questions about the nature of the human mind. Energized by Kanwisher’s enthusiasm for finding out how and why the brain works as it does, her team collaborates broadly and embraces various tools of neuroscience. But their core discoveries tend to emerge from pictures of the brain in action. For Kanwisher, the Walter A. Rosenblith Professor of Cognitive Neuroscience at MIT, “there’s nothing like looking inside.”
Kanwisher and her colleagues have scanned the brains of hundreds of volunteers using functional magnetic resonance imaging (fMRI). With each scan, they collect a piece of insight into how the brain is organized.

Recognizing faces
By visualizing the parts of the brain that get involved in various mental activities — and, importantly, which do not — they’ve discovered that certain parts of the brain specialize in surprisingly specific tasks. Earlier this year Kanwisher was awarded the prestigious Kavli Prize in Neuroscience for the discovery of one of these hyper-specific regions: a small spot within the brain’s neocortex that recognizes faces.
Kanwisher found that this region, which she named the fusiform face area (FFA), is highly sensitive to images of faces and appears to be largely uninterested in other objects. Without the FFA, the brain struggles with facial recognition — an impairment seen in patients who have experienced damage to this part of the brain.
Beyond the FFA
Not everything in the brain is so specialized. Many areas participate in a range of cognitive processes, and even the most specialized modules, like the FFA, must work with other brain regions to process and use information. Plus, Kanwisher and her team have tracked brain activity during many functions without finding regions devoted exclusively to those tasks. (There doesn’t appear to be a part of the brain dedicated to recognizing snakes, for example).
Still, work in the Kanwisher lab demonstrates that as a specialized functional module within the brain, the FFA is not unique. In collaboration with McGovern colleagues Josh McDermott and Evelina Fedorenko, the group has found areas devoted to perceiving music and using language. There’s even a region dedicated to thinking about other people’s thoughts, identified by Rebecca Saxe in work she started as a graduate student in Kanwisher’s lab.
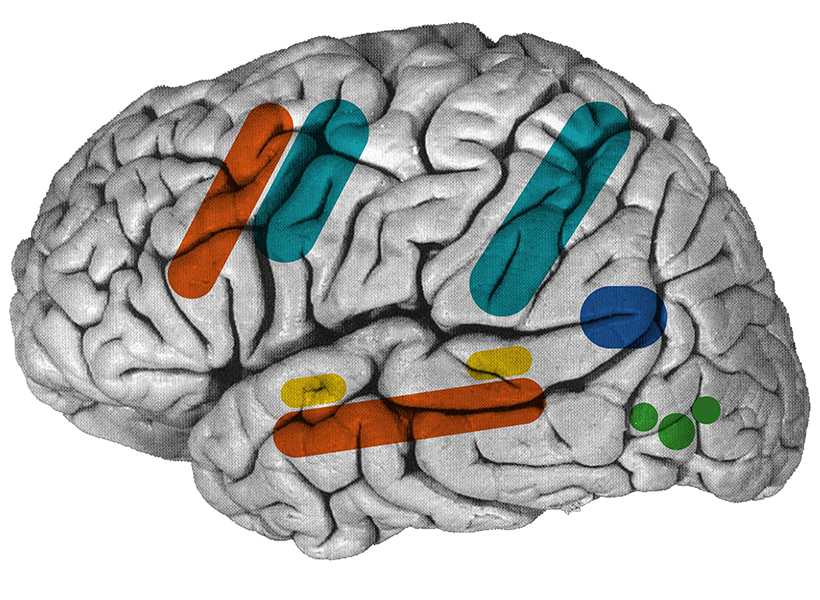
Having established these regions’ roles, Kanwisher and her collaborators are now looking at how and why they become so specialized. Meanwhile, the group has also turned its attention to a more complex function that seems to largely take place within a defined network: our intuitive sense of physics.
The brain’s game engine
Early in life, we begin to understand the nature of objects and materials, such as the fact that objects can support but not move through each other. Later, we intuitively understand how it feels to move on a slippery floor, what happens when moving objects collide, and where a tossed ball will fall. “You can’t do anything at all in the world without some understanding of the physics of the world you’re acting on,” Kanwisher says.
Kanwisher says MIT colleague Josh Tenenbaum first sparked her interest in intuitive physical reasoning. Tenenbaum and his students had been arguing that humans understand
the physical world using a simulation system, much like the physics engines that video games use to generate realistic movement and interactions within virtual environments. Kanwisher decided to team up with Tenenbaum to test whether there really is a game engine in the head, and if so, what it computes and represents.
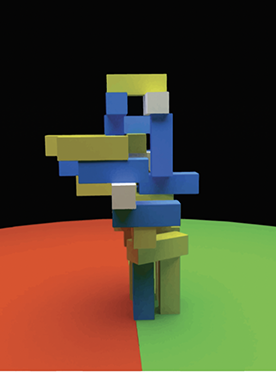
To find out, Kanwisher and her team have asked volunteers to evaluate various scenarios while in an MRI scanner — some that require physical reasoning and some that do not. They found sizable parts of the brain that participate in physical reasoning tasks but stay quiet during other kinds of thinking.
Research scientist RT Pramod says he was initially skeptical the brain would dedicate special circuitry to the diverse tasks involved in our intuitive sense of physics — but he’s been convinced by the data he’s found. “I see consistent evidence that if you’re reasoning, if you’re thinking, or even if you’re looking at anything sort of “physics-y” about the world, you will see activations in these regions and only in these regions — not anywhere else,” he says.
Pramod’s experiments also show that these regions are called on to make predictions about the physical world. When volunteers watch videos of objects whose trajectories portend a crash — but do not actually depict that crash — it is the physics network that signals what is about to happen. “Only these regions have this information, suggesting that maybe there is some truth to the physics engine hypothesis,” Pramod says.
Kanwisher says she doesn’t expect physical reasoning, which her group has tied to sizable swaths of the brain’s frontal and parietal cortex, to be executed by a module as distinct as the FFA. “It’s not going to be like one hyper-specific region and that’s all that happens there,” she says. “I think ultimately it’s much more interesting than that.”
To figure out what these regions can and cannot do, Kanwisher’s team has broadened the ways in which they ask volunteers to think about physics inside the MRI scanner. So far, Kanwisher says, the group’s tests have focused on rigid objects. But what about soft, squishy ones, or liquids?
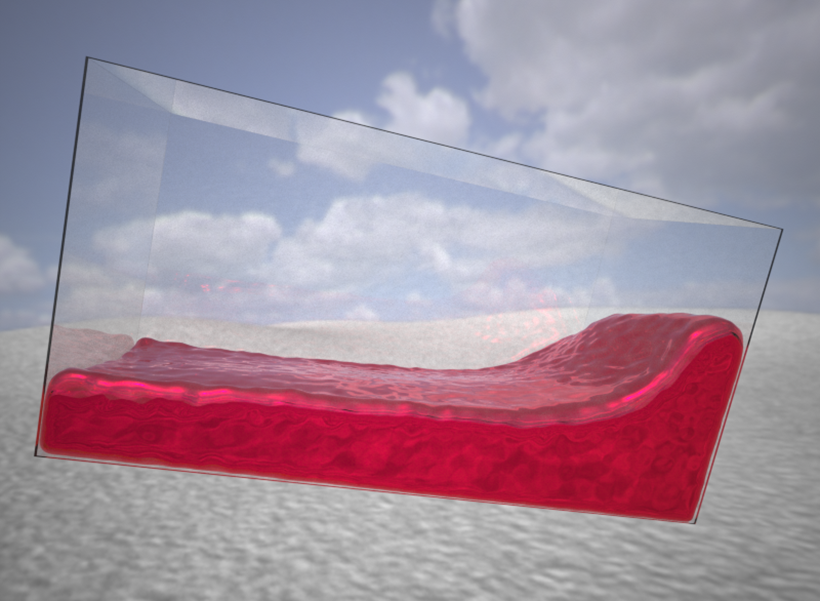
Vivian Paulun, a postdoc working jointly with Kanwisher and Tenenbaum, is investigating whether our innate expectations about these kinds of materials occur within the network that they have linked to physical reasoning about rigid objects. Another set of experiments will explore whether we use sounds, like that of a bouncing ball or a screeching car, to predict physics physical events with the same network that interprets visual cues.
Meanwhile, she is also excited about an opportunity to find out what happens when the brain’s physics network is damaged. With collaborators in England, the group plans to find out whether patients in which stroke has affected this part of the brain have specific deficits in physical reasoning.
Probing these questions could reveal fundamental truths about the human mind and intelligence. Pramod points out that it could also help advance artificial intelligence, which so far has been unable to match humans when it comes to physical reasoning. “Inferences that are sort of easy for us are still really difficult for even state-of-the art computer vision,” he says. “If we want to get to a stage where we have really good machine learning algorithms that can interact with the world the way we do, I think we should first understand how the brain does it.”